Leaving product analytics
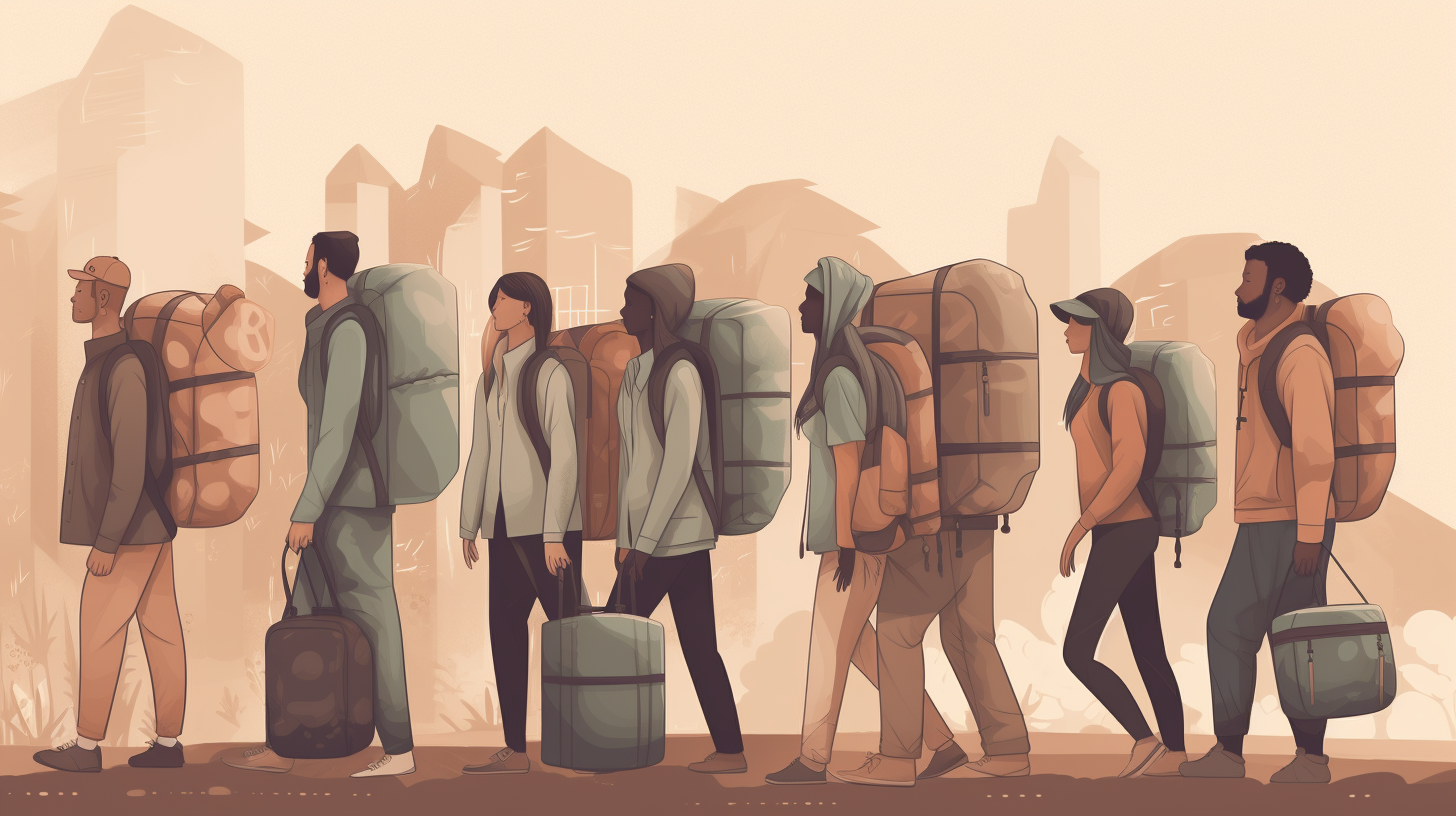
The current situation: Amplitude, Mixpanel, and Heap are setting out to new offerings
Last year Amplitude announced that they officially had left the product analytics space.
https://amplitude.com/blog/new-digital-analytics
Did they call it like that? Of course not. Did they really leave it? Depends on the definition. So let's start there.
What is product analytics (in a nutshell) -
an approach to understanding how users or accounts use a digital product? With a focus on feature usage, cohort analysis, and based on retention. Product analytics is based on event data that is sent when users or systems perform a specific action. (ok, that is really short). But it has the important ingredients that we need in the next steps.
Amplitude introduced several new features last year. But the major one was marketing attribution. It enables you to analyze the impact of a marketing campaign on a specific user (and therefore enables also the design of a marketing funnel that does not stop at signup). It also enables you to group campaigns in specific marketing channels (for me, a core asset in marketing analytics). Finally, it gives you different attribution models you can apply and use.
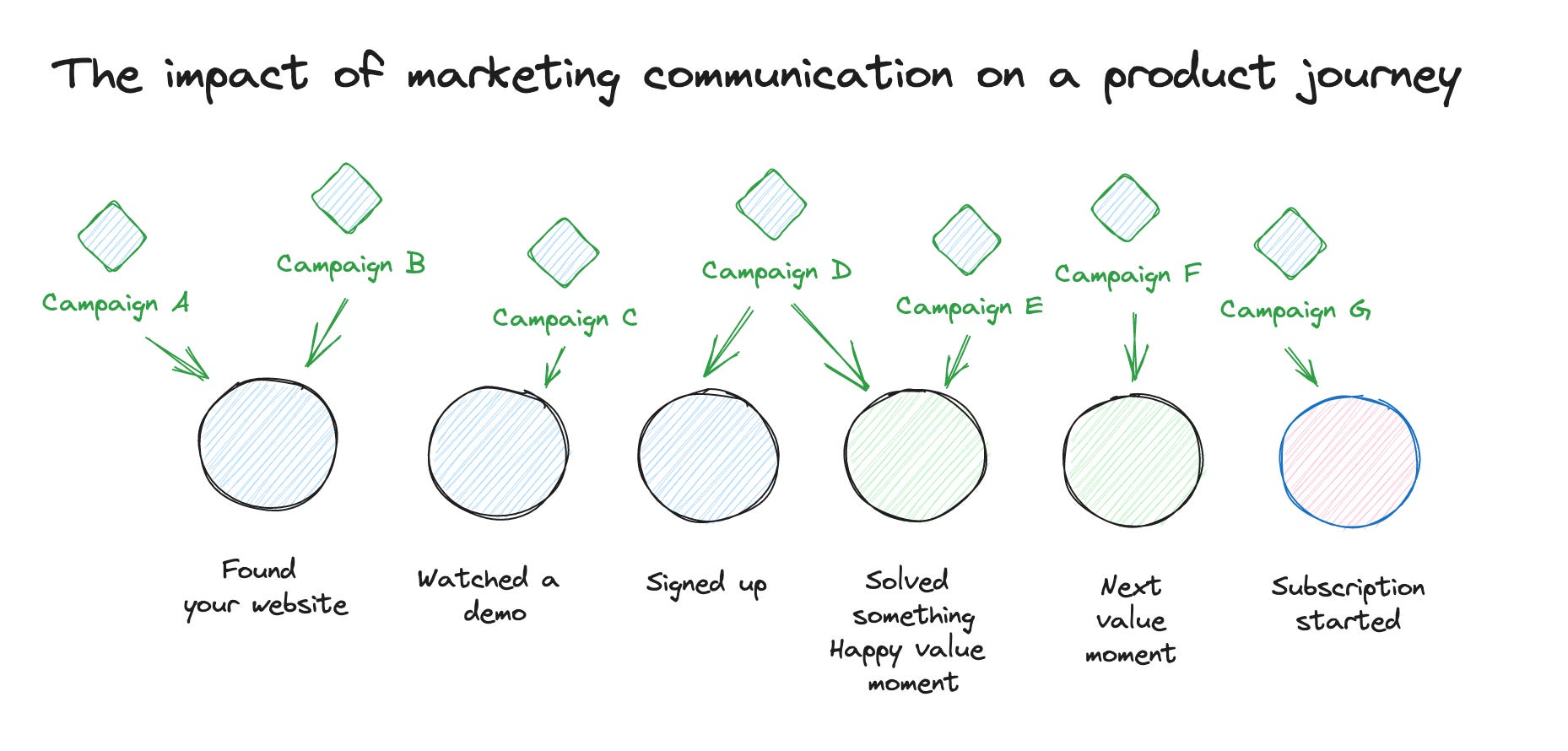
This was a missing core feature for me in the past when I was talking with growth teams, since they need both parts: analyze the product usage performance and where the user came from and how many touchpoints they had during their lifetime.
This use case is clearly beyond classic core product analytics, and it is something we usually call marketing analytics. So Amplitude offers both now, and you can combine them.
Mixpanel introduced similar features just four weeks ago:
https://mixpanel.com/blog/mixpanel-marketing-analytics/
Heap, in comparison, did a realignment of their strategy at the same time as Amplitude but went off to a different direction. Heap's core distinctive feature was always the auto-tracking of basically all interaction in the front end. They sold it as product analytics without implementation. But it sounded easier on paper than in real life since the real juice is in the context that you put into properties, which often can't be read from the browser context. And Amplitude & Mixpanel, plus the league of analytics consulting, were not tired of preaching that auto-tracking is the work of evil forces. They were not totally right, but they were definitely loud enough.
So Heap rethought their whole approach and found an interesting direction. On the one hand, due to the huge amount of automatically tracked data, they could discover usage patterns in the front end that you were unaware of. That is quite interesting.
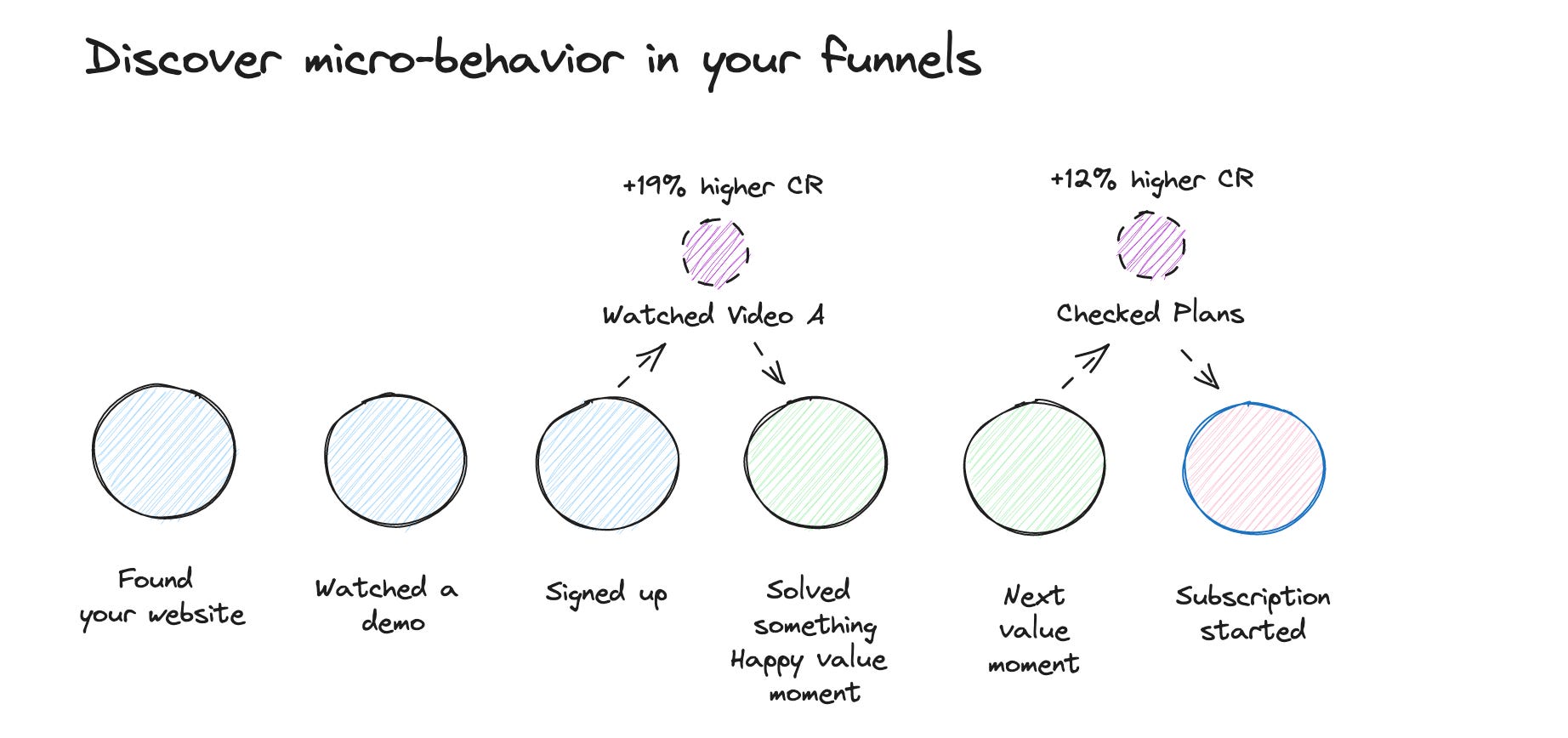
Additionally, they combined event data with mouse tracking and survey data. Therefore enrich the analysis workbench significantly.
They don't call themselves like that, but for me, they just created a new analytics category of user & customer experience analytics. More on that later.
Posthog tried a broader approach from the start. Significantly later to the product analytics game, they tried two significantly different approaches. First, they target a different ICP: the product engineer. They do it by making Posthog more transparent (there is a minor open-source version) but making the whole setup more extendable with plugins.

And second, they call themselves the product OS. This mostly means that they offer a collection of useful things to improve your product that the other tools only offered as expensive add-ons (or not all): Experimentation, Mouse tracking, and group-level analytics.
And we have new ones arriving at the shores with Kubit and Netspring.
Kubit and Netspring are trying to solve something I have been waiting for for years now. Product analytics on top of your events in your data warehouse. No more weird data loadings and enrichment (where most of them never worked). And mostly, no two setups for classic BI and product analytics use cases. We spent some more time with this approach later.
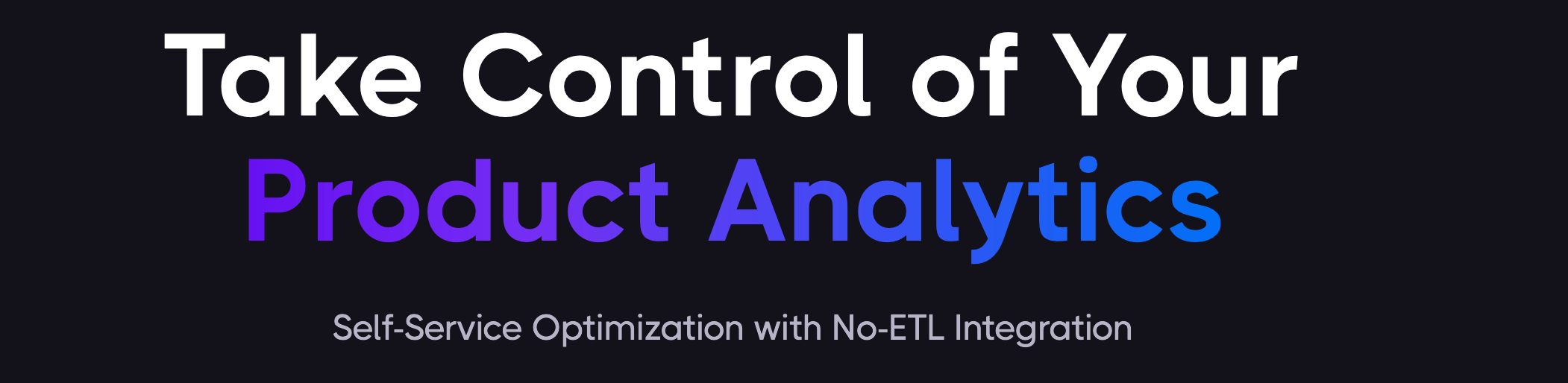
Thanks for reading the hipster data stack! Subscribe for free to receive new posts and support my work.
Some history of product analytics
An interesting fact about Amplitude and Mixpanel: both started as mobile analytics solutions (to be fair Mixpanel offered both app and web but was very popular for apps).
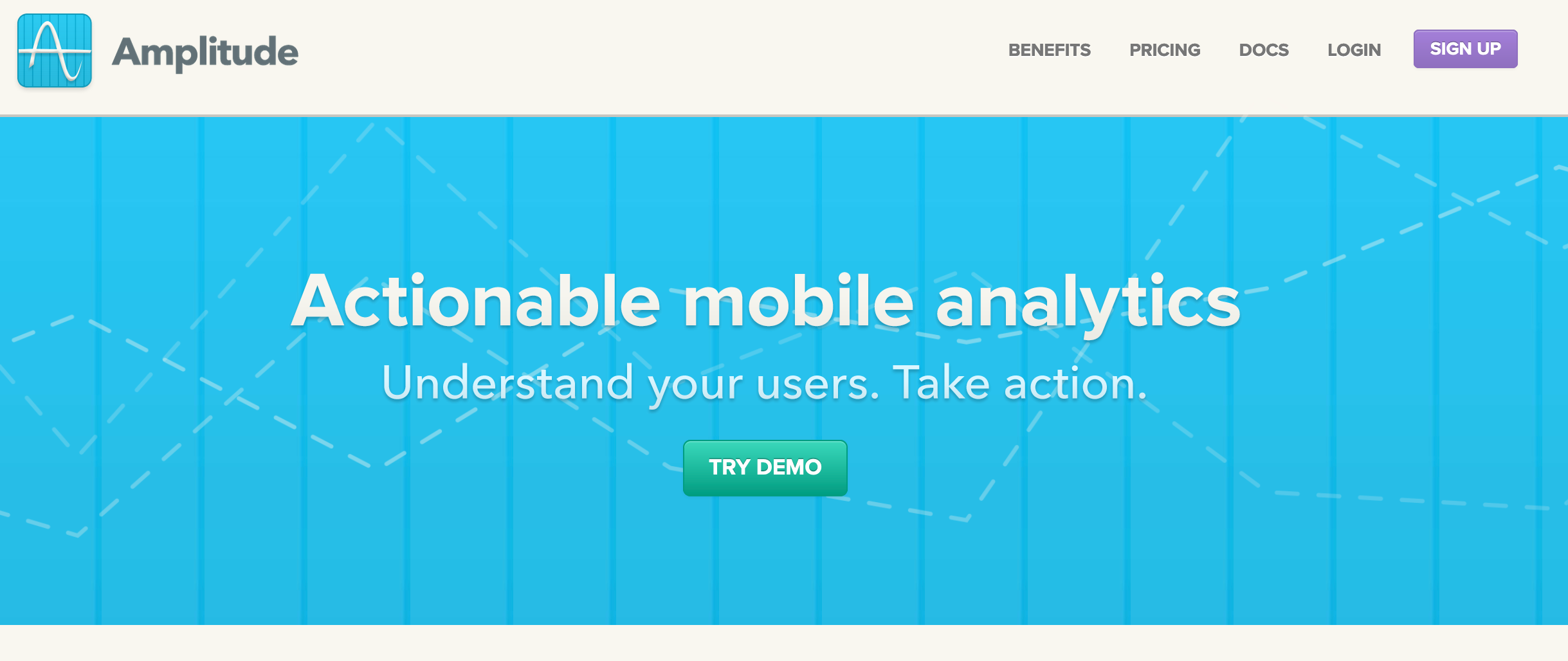
Interestingly we have seen something similar in the past already. Some of you might remember that Amplitude and Mixpanel both started out as mobile analytics solutions. Over time, both also added a web SDK which could be used for websites and web applications. But there were no words about product analytics at all. Even when both tools already had the core ingredients you need for proper product analytics already: funnels, cohort retention analysis, and event data tracking. And mobile brought something else - a pretty consistent identifier: a core asset to product analytics work.
And then came Firebase Analytics
Firebase was initially just a backend database that was used for app development due to its easy real-time capabilities (quite impressive at that time). Then Google bought Firebase and positioned it as a backend service for mobile apps. This also included a brand new mobile analytics solution at that time called Firebase Analytics. And you got all for free (well, we know not really for free, but who cared at that time).
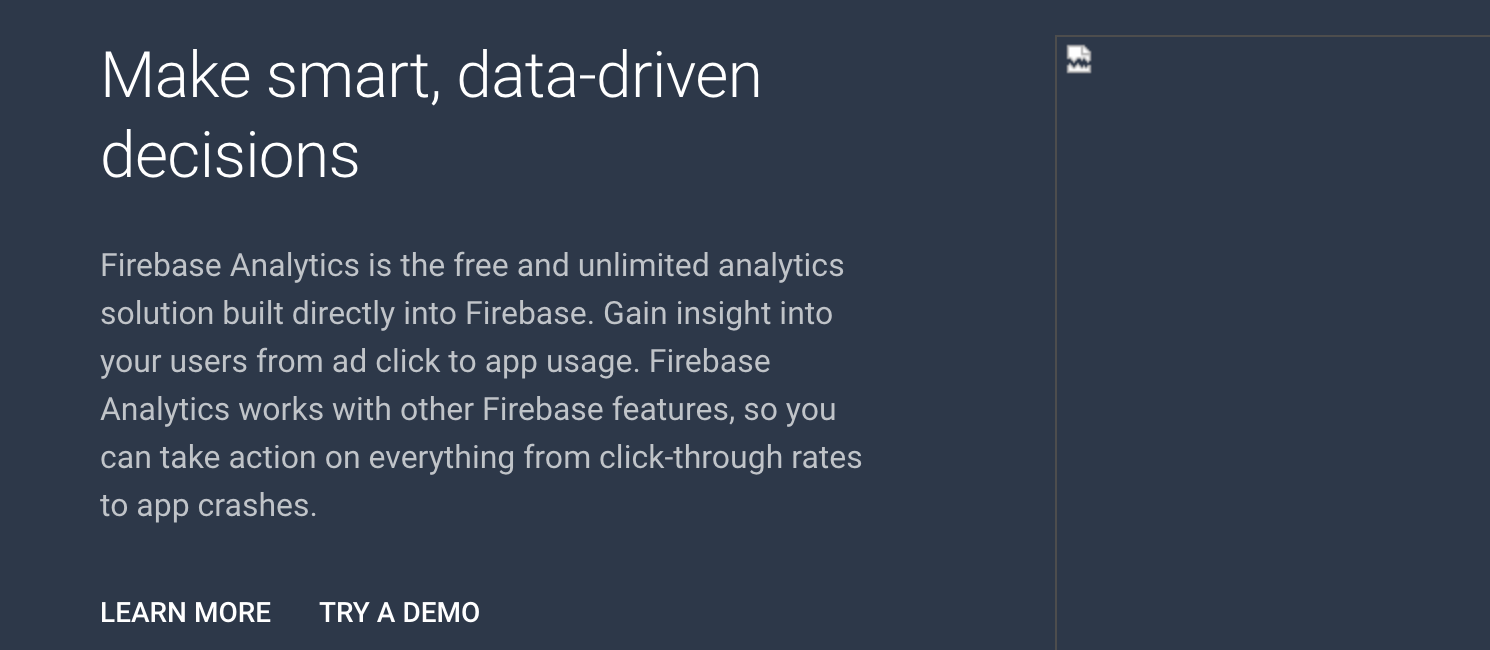
And Firebase Analytics (and Firebase) took the same path that Google Analytics went before—gaining market share quickly and significantly. Firebase analytics did not have as extensive features like Amplitude and Mixpanel, but for most people, it was sufficient (also similar to Google Analytics). So the whole market became more difficult.
And mobile never really caught fire for Analytics
But there was a different problem in the mobile analytics space, which will already foreshadow what we will see in the product space.
Attribution problem
The major use case for analytics for a website is for marketing and analyzing their campaign performance. This is also the core use case for analytics for a mobile app. But here it is more difficult. When you run a paid campaign for mobile app installs, there is always an App or Play store in between. Since you don't control it, you lose any campaign information in between. So by default, when someone clicks an ad, installs the app, you load the app and want to track where the user was coming from, you can't because there is no information.
That creates a small selection of special analytics tools called mobile attribution tools. They remember the device id when someone clicks an ad and then check this device id against the one you send to them after an install and then tell you the campaign (that doesn't work so easily anymore after iOS 14.5, but even SKAdnetwork can be handled via these tools). So this becomes the essential tool for mobile analytics, at least for the marketing team.
Product not data-driven?
Now get to a mystery one. Throughout my data career, I tried to convince, motivate and push product teams to use event data as an input driver for making product decisions.
Most of the time, I fail.
To exaggerate: Product teams are the most quantitive data-avoiding teams I know. And to be honest. I don't really have an explanation for it. It is a post by itself to investigate it. So, for now, based on my experience and the experience of plenty of data people, getting product teams to work with product analytics is a tough job.
Thanks for reading the hipster data stack! Subscribe for free to receive new posts and support my work.
So, why move on?
Why do you move on with your product?
Moving on mostly means that your ICP (ideal customer profile) changes over time. That is quite a natural process. You usually start with a small and narrow ICP definition because this makes the product design significantly easier. Then you will start to attract audiences beyond your ICP. And some of them might interest you because they have slightly different features but are still close enough to your core product. So moving on is a natural process and sometimes not really visible to customers.
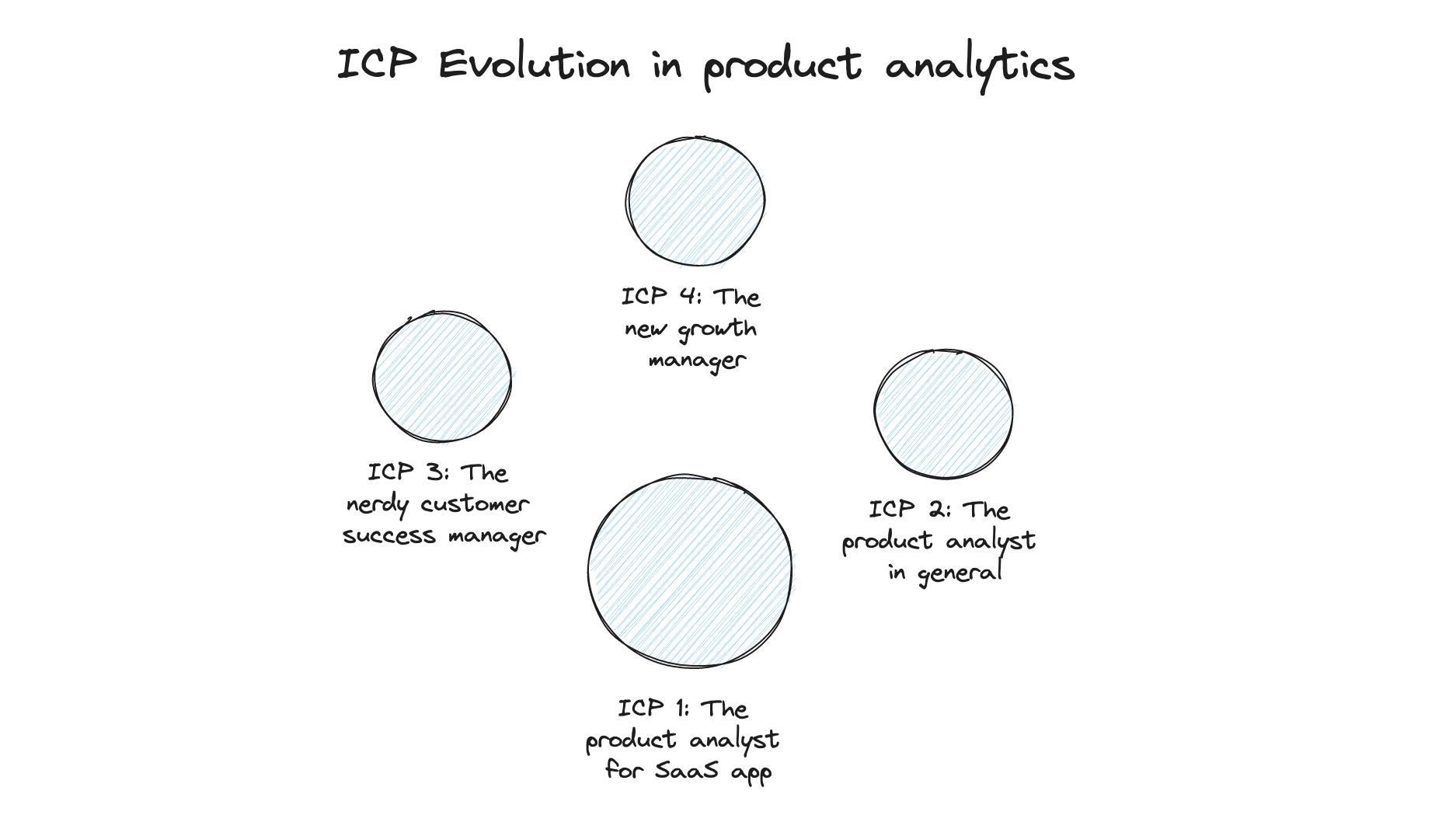
But what Amplitude and Mixpanel did is a visible move. Yes, you can still read product on their websites (which is important), but Amplitude now calls itself a digital analytics platform, and Mixpanel speaks of analytics for everyone.
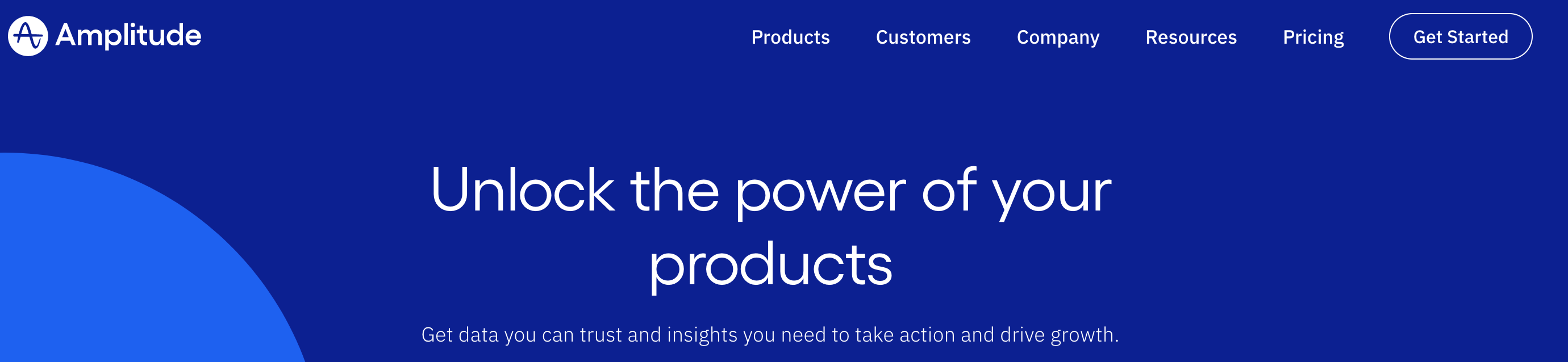
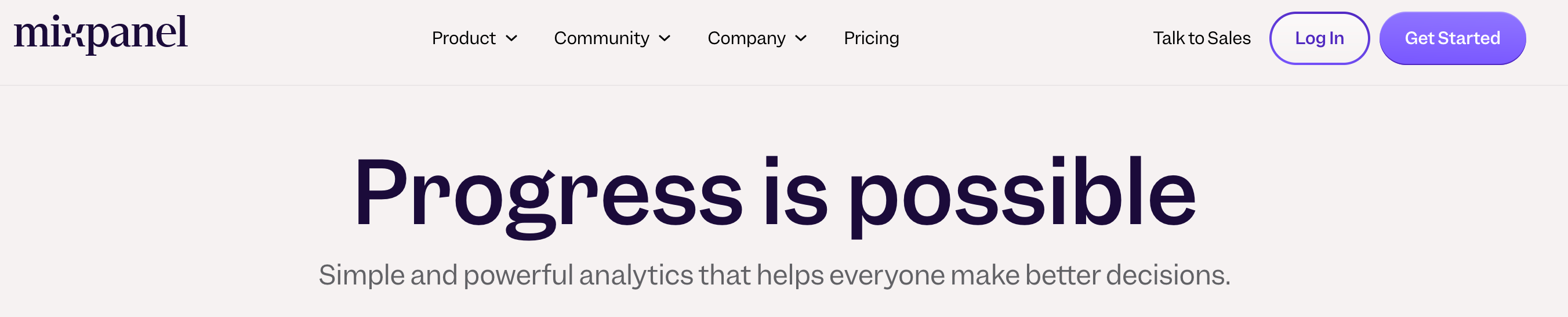
Let's have a look if some significant forces could have forced this evolution.
Product teams are difficult customers, and Growth teams need more.
We mentioned this already above; from my experience, product teams are the hardest to work with regarding analytics data. They are quite confident to work with qualitative data like interviews or surveys. And this might already be a part of the answer, which is why it is so hard to work with them. You can develop a good product by simply working only with qualitative data. The feedback cycles might be longer, but that does not have a bad impact at all.
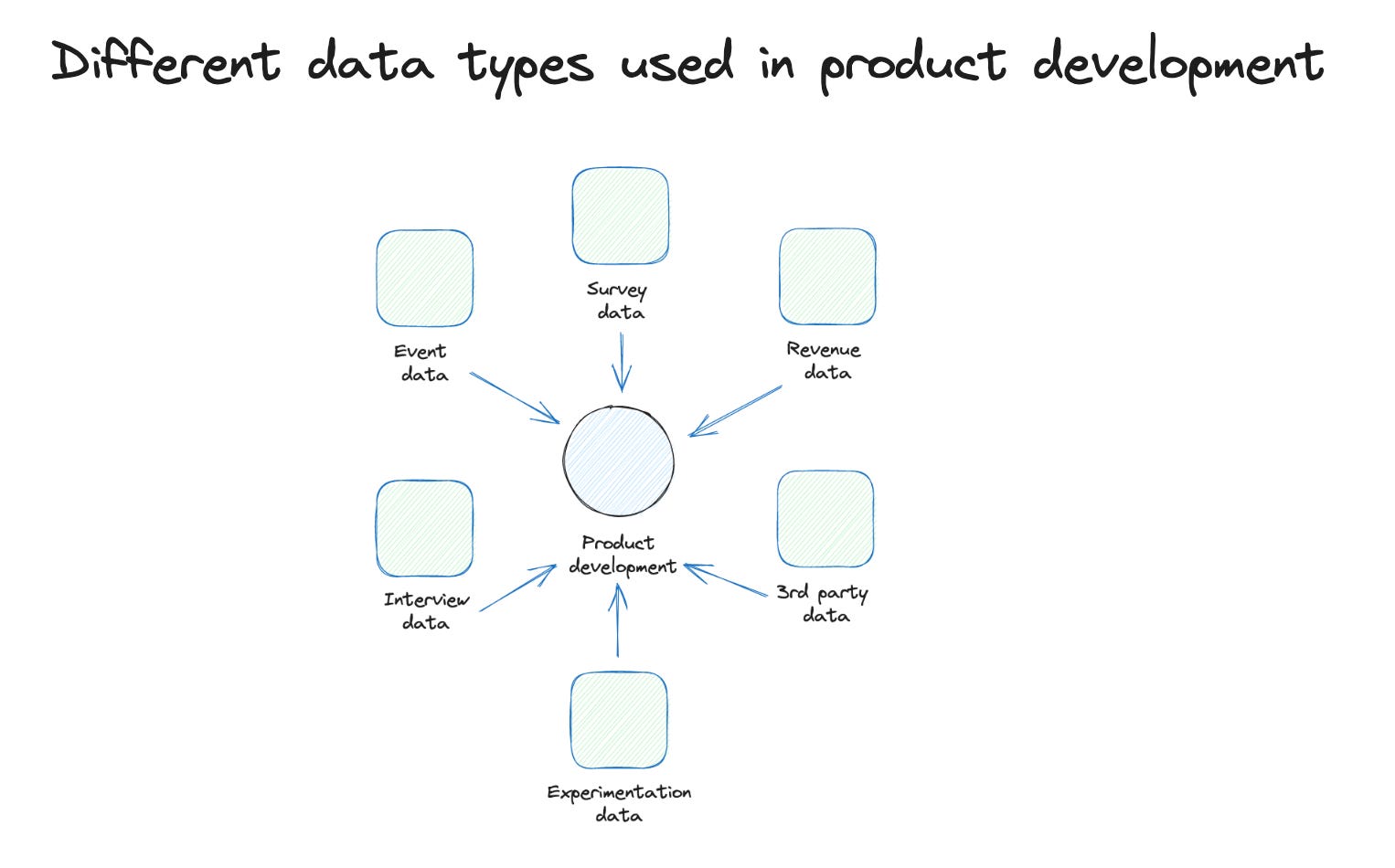
Event data in products serve two main jobs: fast feedback loops and unbiased feedback.
Fast feedback loop means that you can get quick indicators (after hours or 1-2 days) of how a new feature is developing (usage data) and, after a week, how it impacts the general performance towards your goals. You can get similar data with qualitative measures but this usually needs more time and effort.
Unbiased feedback because the data is collected while your users are in real use case situations. It's never a lab environment or any environment at all (what you have in a survey). This can give you perspective on the data that might differ slightly from your interview data.
So event data is useful for product teams but most of them still struggle to make heavy use of it. Another reason: it is far more complicated than marketing analytics
Marketing analytics is straightforward. You define your customer journey funnel - which is usually defined by 5-7 core events. You track them and ensure all marketing campaigns that link to your website have tracking parameters. And then, you analyze the campaigns across this funnel. Your main concern then is how you handle attribution (to be fair that can be a real pain).
In product analytics, there is no simple funnel. Yes, you will also use the core customer journey funnel as a baseline since later steps usually happen within the product. But a core optimization metric for the product is how users return since most products nowadays are built on subscriptions. For this, you need to understand how cohort analysis works and this is quite a complex topic to get your head around
But a new ICP is appearing on the horizon: Growth teams and even better ones focusing on Product-led growth. They have some roots in product development, but their main focus is on growing the user base and, finally, the subscription base of a product. To do this, they need fast feedback loops and are naturally more metrics-driven than classic product managers. But they need something that classic product analytics could not offer: marketing attribution. We cover this in a second.
The Google Analytics opportunity
And then there the Google Analytics opportunity, but maybe it was just a coincidence. Almost 14 months ago Google announced that it will sunset the Google Analytics universal version and forced all users to migrate to Google Analytics 4. This is not a "click a button" migration, it is a full "implement new code" migration. So it takes a lot effort to achieve it.
And then, Google decided to make Google Analytics 4 a different product. The different data model was necessary, but they also significantly changed the UI and data modeling. This leads to and is still leading to a lot of confusion and frustration in the GA user audience. No one really knows for whom the solution is built now. Google Analytics was always the best out-of-the-box tool for marketing analytics. You can still do similar things but it does not feel out-of-the-box anymore.
As reminder, here is my video about the weird Google Analytics 4 user strategy:
There is an opportunity for products to offer an advanced version of Google Analytics with a consistent product design. Something in between Google Analytics and Adobe Analytics.
Big GA accounts are paying 100k+ for their setup, so there is a budget available for different solutions since they have to migrate. And also, the deep Google Ads integration is not the biggest asset anymore since marketing channels were diversified more than 5 years ago.
Analytics for all
And we may see again a time when it makes more sense to have one analytics product for all teams. For quite some time, I recommended multi-product setups. Just because there were specific marketing needs (like marketing campaign attribution) and special product needs like cohort analysis that you couldn't get with one product. So we ended up with something like Segment or Rudderstack for event collection and then Google Analytics and Amplitude on top of this data. Not a bad solution but with plenty of trade-offs.
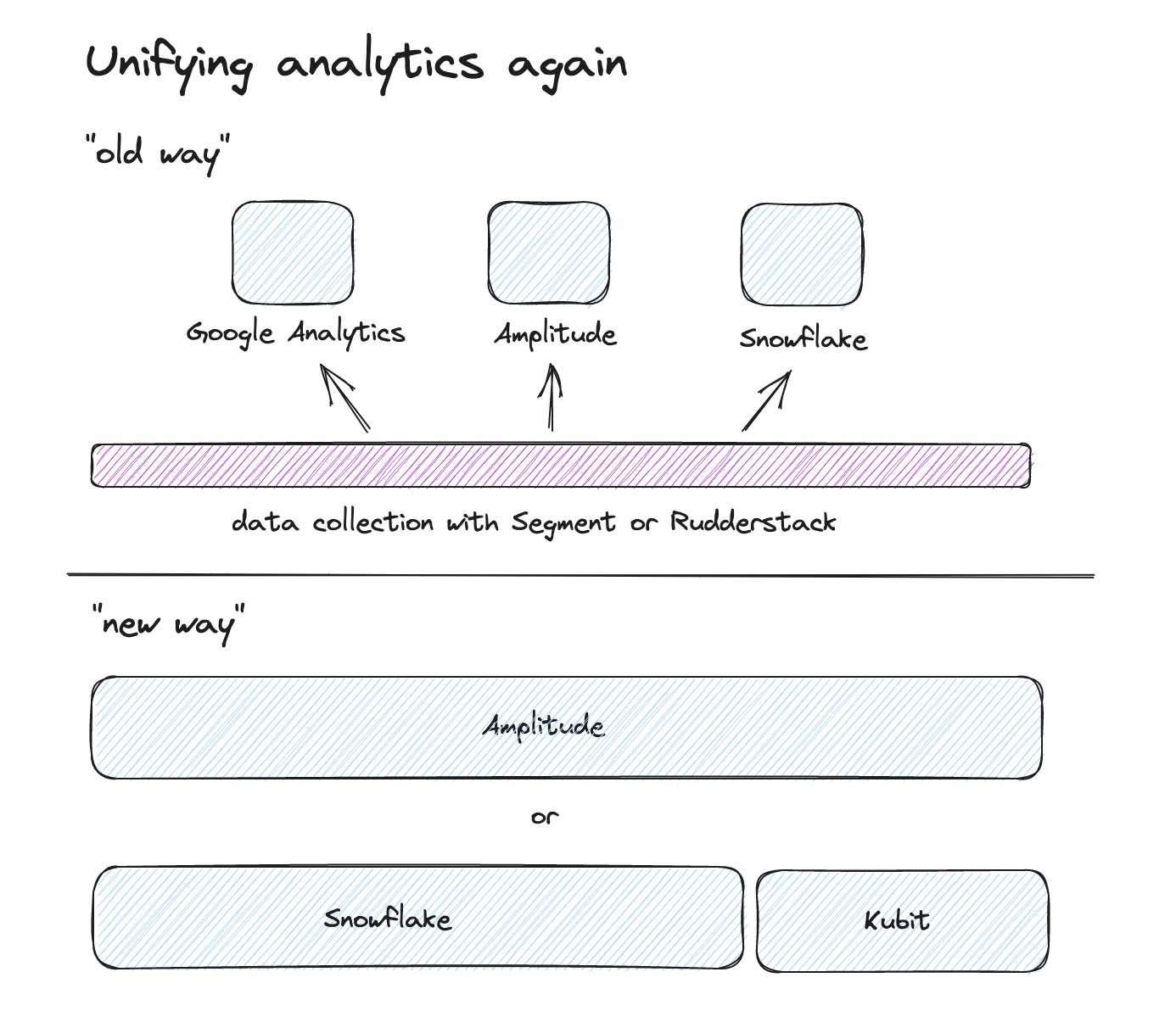
Having all things about customer & product events in one product can enable users faster and prevent complex onboardings and trade-offs. Therefore there is a growing market for a one-for-all analytics solution. We get to this later, with new requirements are already coming up.
Thanks for reading the hipster data stack! Subscribe for free to receive new posts and support my work.
Where are Amplitude and Mixpanel going?
I will describe what has changed based on Amplitude's implementation since this is the one I have tested and applied.
So what has been added that makes it a different platform:
Marketing campaign attribution
Even when it sounds simple, this core feature was missing before. Because it is not so simple. It means two core concepts:
A user can have multiple marketing touchpoints. These touchpoints are usually the user's information when accessing the website or application, like UTM parameters or referral data. When you look at a conversion goal like a subscription, you want to have a proper way how to attribute these to all the marketing touchpoints this account (yes, I meant account instead of user here) had before they signed up. This can help you to analyze what kind of communication has an impact on the customer journey.
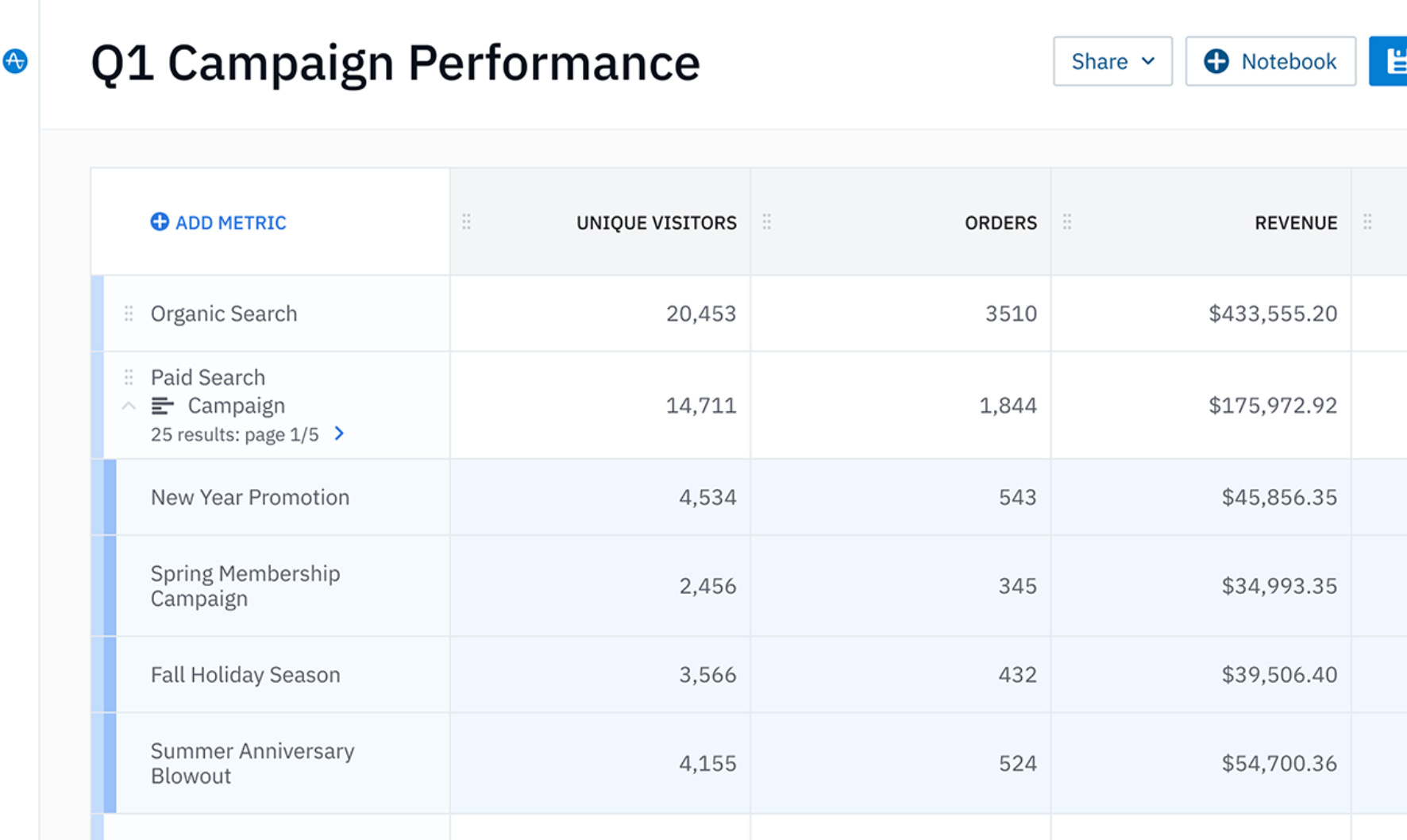
Something that is often overlooked is the definition of marketing channels. Analyzing on the campaign level can be tedious, especially when you run plenty of campaigns. Therefore you can group campaigns into specific channels. You choose these channels based on similar kinds of users and behavior you might get. So it makes total sense to group remarketing campaigns into a remarketing channel even when they are on search because they usually have different performance than normal search campaigns. The same goes for brand campaigns.
Data tables
Maybe the most overlooked new feature. But it is such a powerful one.
Marketing campaign performance reports are usually not really some charts; the real juice is a big table with all campaigns, the funnel performance, attributed conversions, and a potential cost/revenue relation, and this, in the best case, over time. Think of it as a huge pivot table.
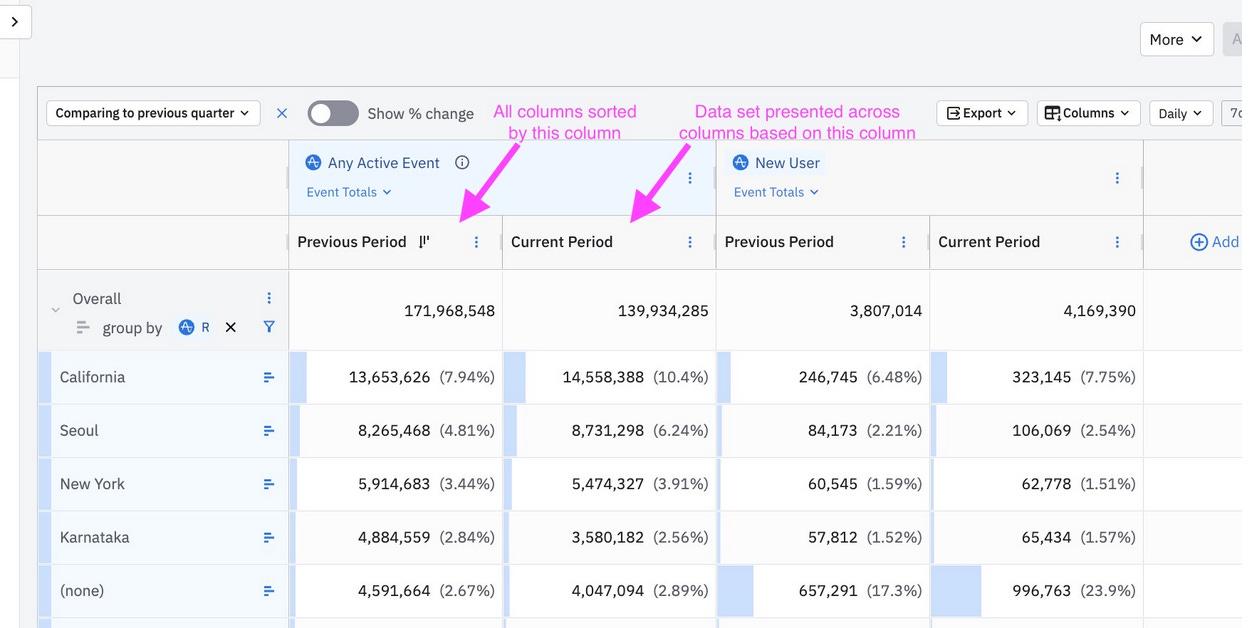
This kind of feature was missing in Amplitude (and, to be honest, also from GA - yes, there are custom reports, but this is a light version of what I am talking about).
The new data tables are extremely powerful and not only for marketing campaign analysis. This would be a new post to describe them.
Cost attribution
When discussing marketing conversion attribution, you will next discuss cost attribution. In the end, you spent plenty of money on marketing campaigns, and based on the conversion performance, you might like to establish an ROI or a cost/revenue metric to see at one glance if a campaign is problematic and needs attention. Getting cost data into Amplitude before was not possible and it was always a step to export Amplitude data and then hook it up with cost data.
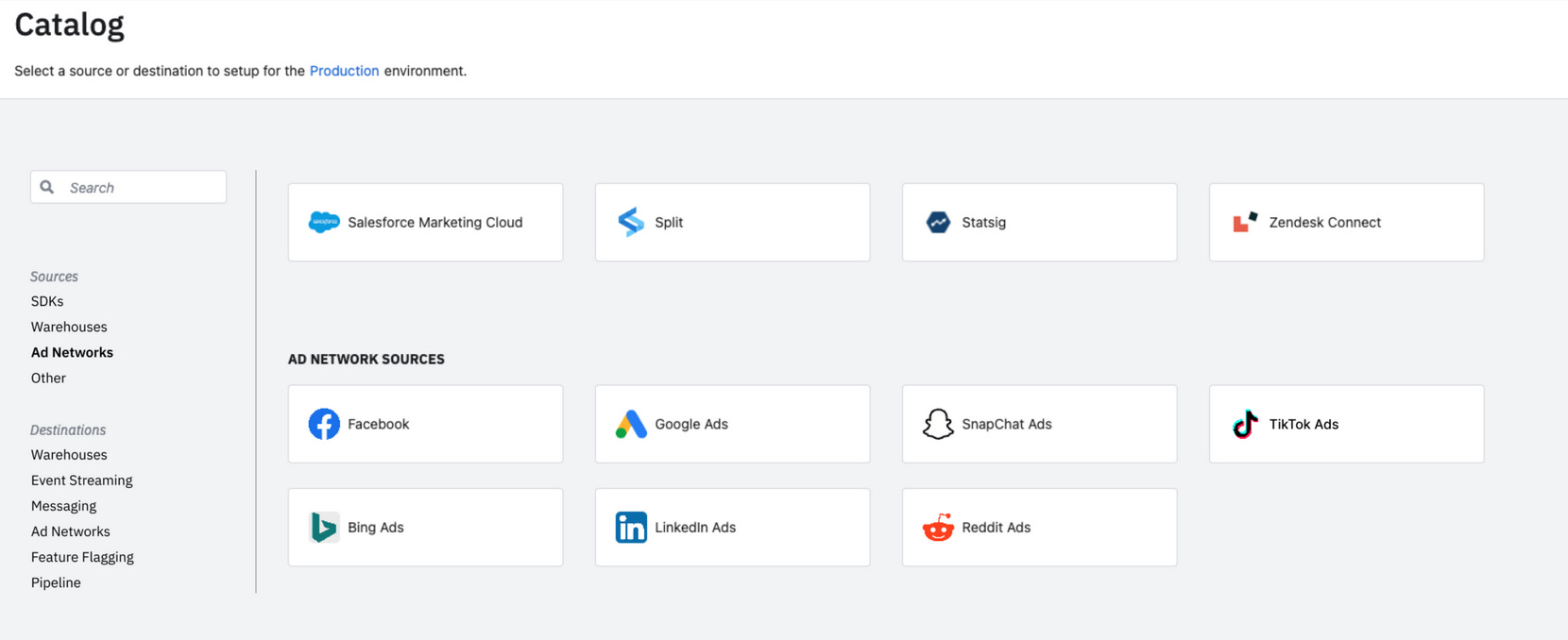
Now you can import specific marketing cost data automatically into Amplitude and work with it.
These features now enable growth teams to work with a tool like Amplitude since it provides the missing pieces of the former puzzle. But it also makes Amplitude a pro tool for marketing analysts since Amplitudes funnel and cohort analysis and even data tables are more powerful than the Google Analytics version.
E-Commerce Power Analytics?
The interesting part is what Amplitude can do in the E-Commerce analytics space. E-commerce analytics was always an enhanced marketing analytics use case for me. And again Google Analytics was always the best out-of-the-box solution for it. E-Commerce analytics requires all the possibilities we described above but additionally has requirements towards the data model. One is properly handling object arrays since you usually have multiple products in a cart. Other is specific product attributes that behave like user attributes but are bound to a product. And you might like to have an internal attribution for onsite merch campaigns.
You also need a product perspective. Tools like Amplitude have by nature a user perspective. But you want to analyse how a product is performing across the funnel.
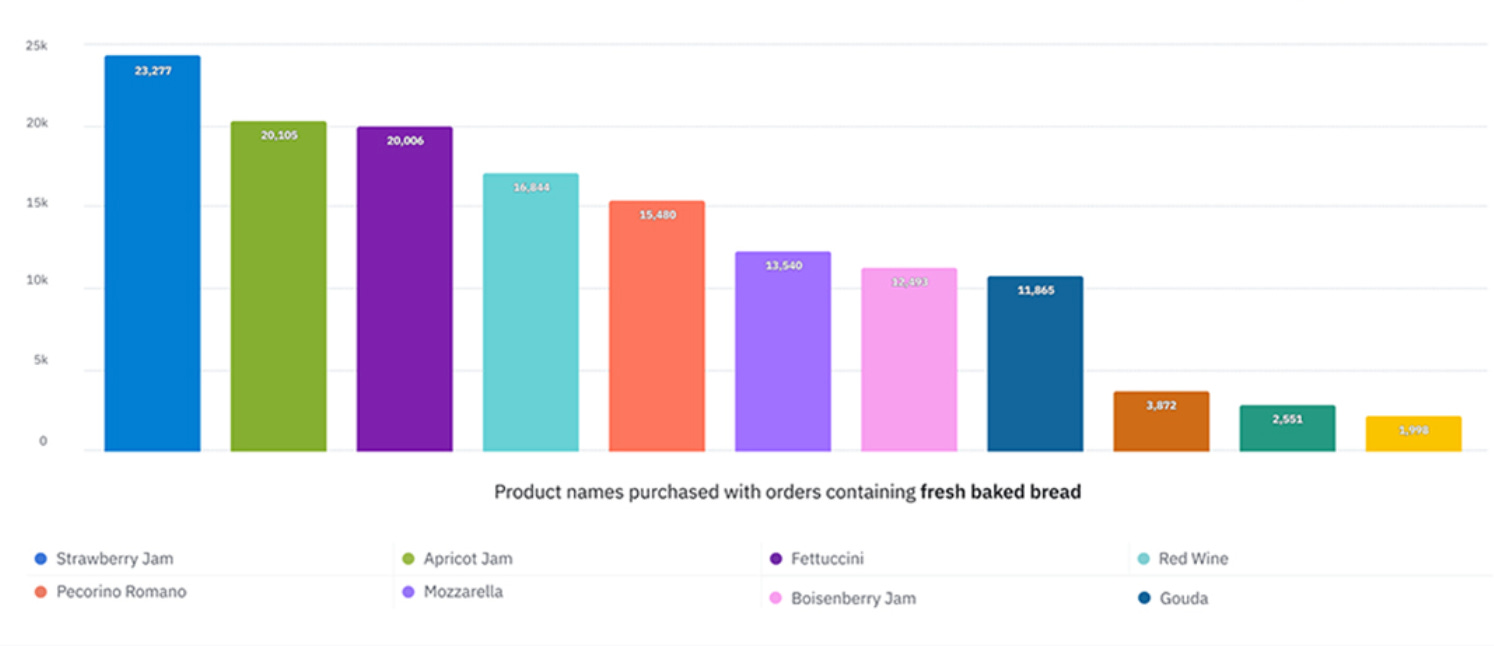
With Cart Analysis, Amplitude is taking a first step into e-commerce-analytics. I haven't tested it yet, therefore can't tell how extensively it already works.
https://amplitude.com/blog/cart-analysis
Where is Heap going?
Entering UX & CX analytics.
Heap took a slightly different direction. Because Heap's core asset was always the auto-collection of events, they have an interesting asset. Additionally, they extended their product with acquisition and added two essential things: AI-based insights and Session Replays.
The AI-based insights can surface interesting user patterns you were unaware of (since they capture every click or touch). One example is that you set up a classic funnel and Heap will recommend you to add or remove specific steps because of the additional data they have collected.
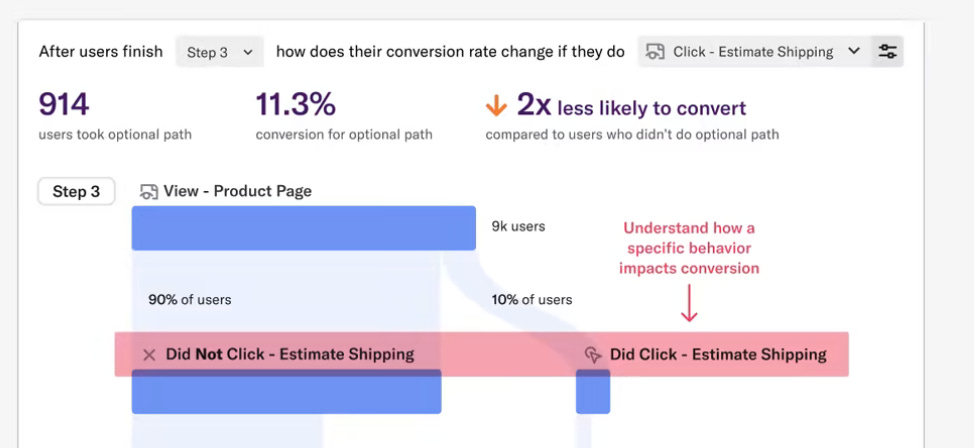
Session replays are also undervalued. When I worked in product, my data stack was always at least a product analytics tool and session replay tool. I then had to make sure that ids are exchanged between both so that I could make connections. The workflow was often like this: I discovered an interesting set of users (like some dropping off in a funnel), I went to the user explorer, picked some user ids, went to the session replay tool, looked for these sessions, and often got interesting hints what was causing the drop off by watching the replay.
In Heap you can now do this just with one click. This is extremely powerful.
You can track events in Heap from all different places (like from your backend) but with their strength just described here, they are a tool in a new kind of category which I call User Experience analytics (or just UX Analytics), which is frontend-centric. And it is a powerful and important one. I would say UX designers are the most under-served group with qualitative data. And from my project experience, they are all eager to get more data. I see shiny eyes when I show them how features are used in detail and how a session replay discovers a flaw in user interaction.
What awaits us with the new arrivals? - Product analytics on your cloud data warehouse
I will focus here on Kubit since I only have experience with this product.
There was a constant itch for me when I worked on data setups. We were defining data setups where we already knew that there would be a time when we have to move on. We always were running at some time in use cases where we had external data that we would need to push into Amplitude. But this was not an easy process and sometimes not possible. So we pulled some data from Amplitude into the data warehouse and ran some reports and analyses there. This brought us two systems to work and analyze the data. I was not really happy with it.
But there was not really a way around it. But one thing kept spinning in my head: why can't I get a product analytics tool that works just on my data warehouse event data?
I wrote about why you can't use BI tools to work with event data here:
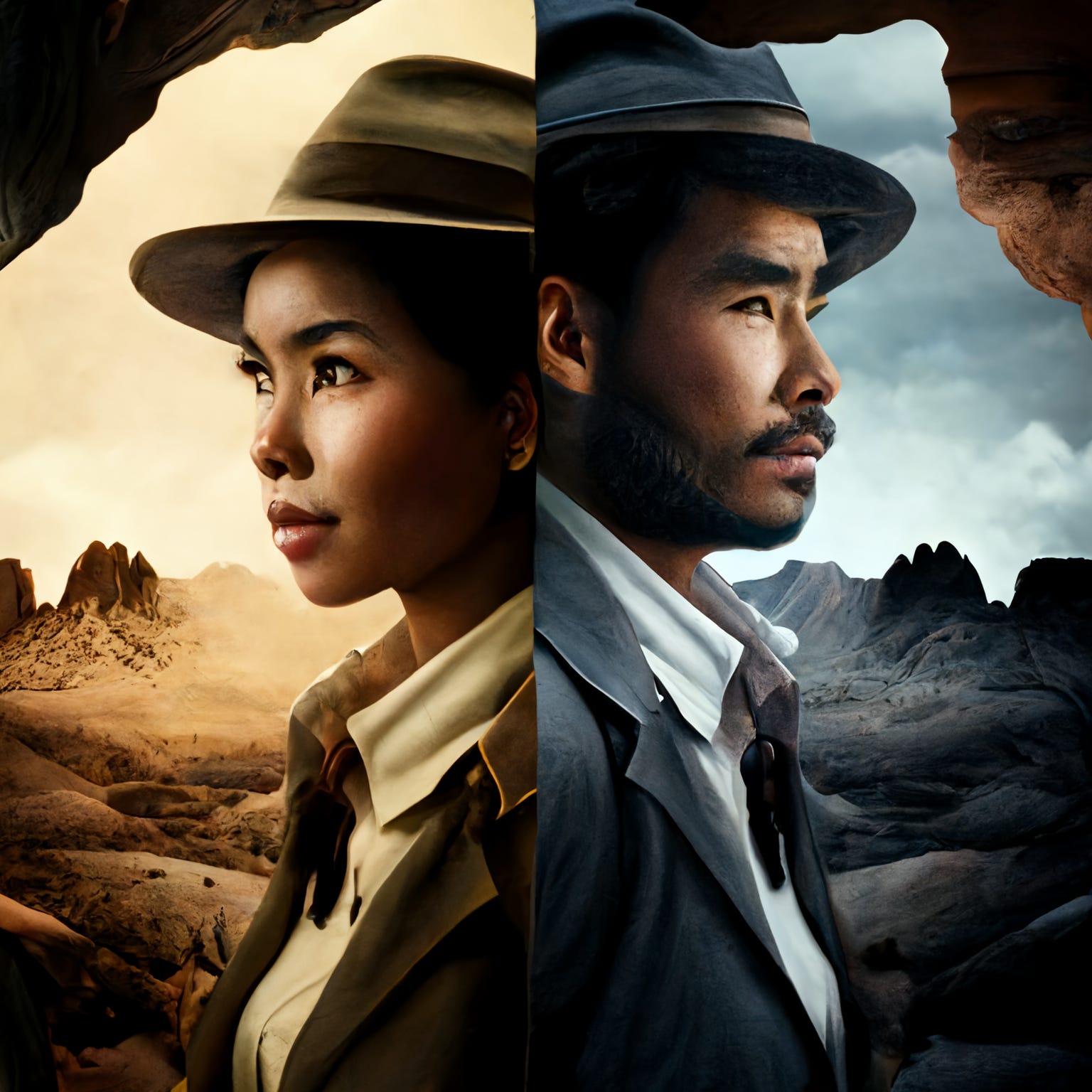
Then I discovered Kubit. And they promised exactly what I wanted. Event analytics on top of my data warehouse data. And for me, this is the future I want to live in.
But as a disclaimer, it is not yet the world for everyone. If you don't have a data team that can load, transform, and prepares the data for Kubit, this is not your setup yet. And it is not as deep as Amplitude is when it comes to the analysis features.
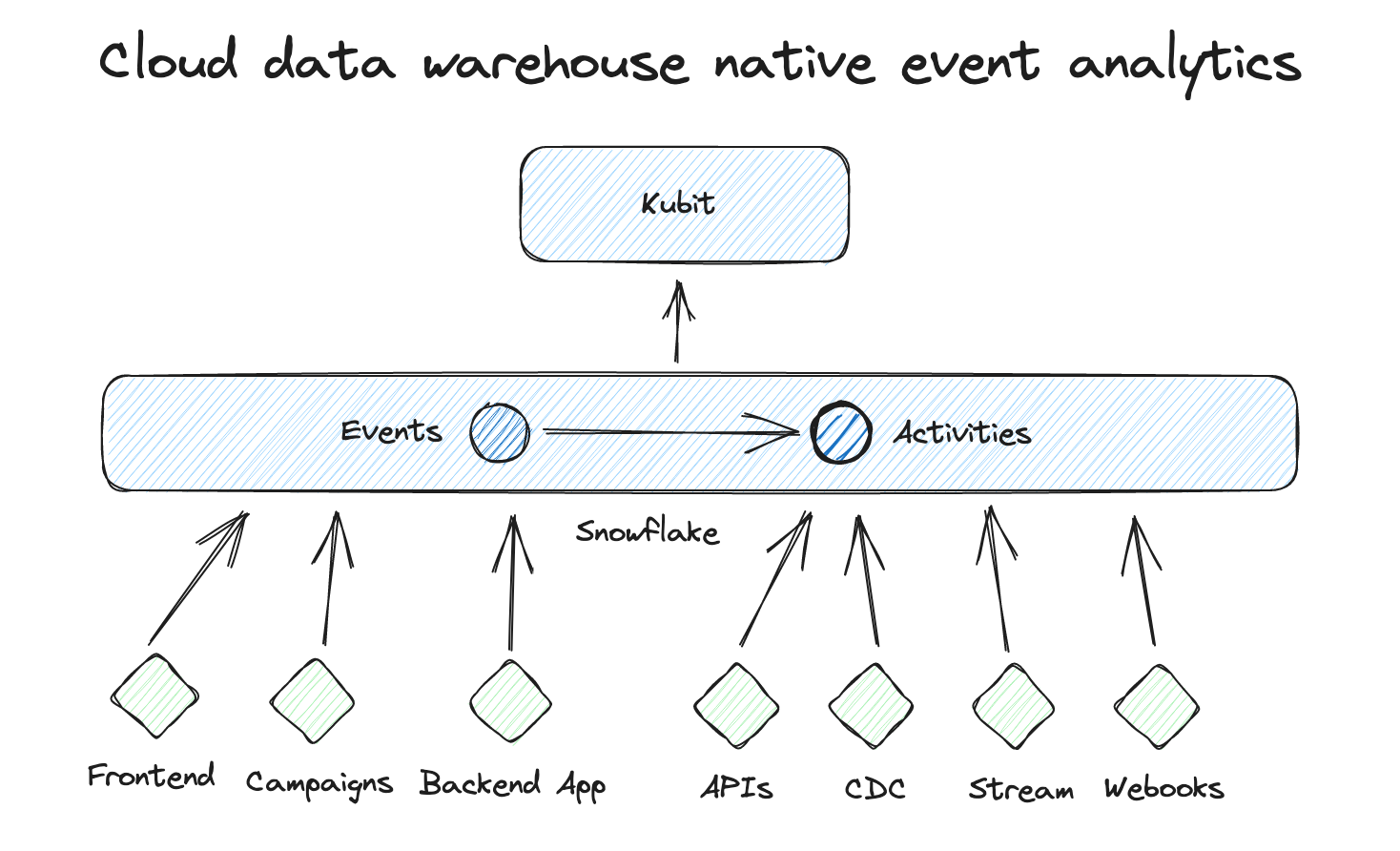
This is not even just product analytics on top of your data warehouse. When we project that approach to the near future, I call it technically event analytics or, more sophisticated: business and product activities platform - or many just activities analytics.
Due to the data warehouse, I can collect and load events from anywhere: my frontends (maybe less), my backend applications (maybe more), directly from databases (CDC), directly from third-party apps via webhooks or I can connect to a data pool of a tool I use and load the events directly from there.
Based on all these different events, we then model activities by selection, transformation, merging or enrichment of the event data. And this data is then used by Kubit to analyze it: All use cases, no limits.
An outlook
It is nice to see that a category that was quite static for some years is opening up and changing quite drastically. Are these changes good news? I think yes. We get features that were missing for quite some time and therefore, there are more use cases we can do. Amplitude manages the new features in a way that is not overwhelming for beginners since they are clearly pro features. Kubit is opening up a new category for mostly data teams who don't want to sync their data to an external tool for analysis.
But new horizons and similar problems:
Event data collection - But we still need event data. So the usual problems with collecting and tracking these stay the same. Data exhaust in, no insights out. One of the reasons why I decided to rename the book I am currently writing from "how to fix your tracking" to "how to collect and track event data". Because I strongly believe that event data will become even more important in the future.
Making sense of data - Just because we have more ways to analyse the data doesn't mean we get more value. The people working with the tools still have to generate this value for the business and product. The pros will get more tooling now to deliver new insights and you still have to train people to become pro - no changes here.
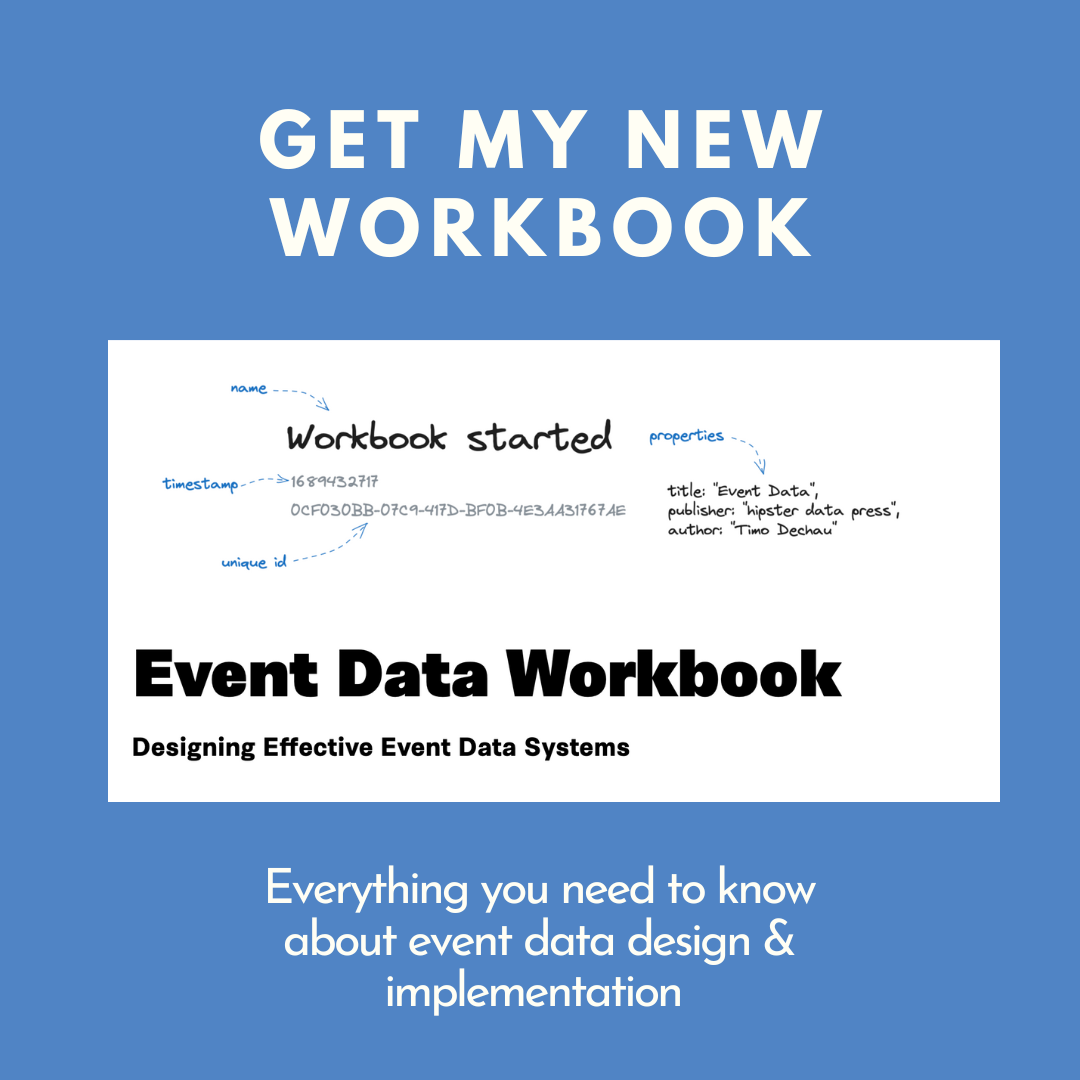