Retention Analytics - the definitive guide
One of the reasons why I like to work on product analytics projects is that some questions are easy to answer.
Here is a regular one: What should be my North Star metric?
And here is my answer that fits in 90% of all cases: A specific retention rate. Like the first-week retention rate for your free users. Because you just need to love users coming back.
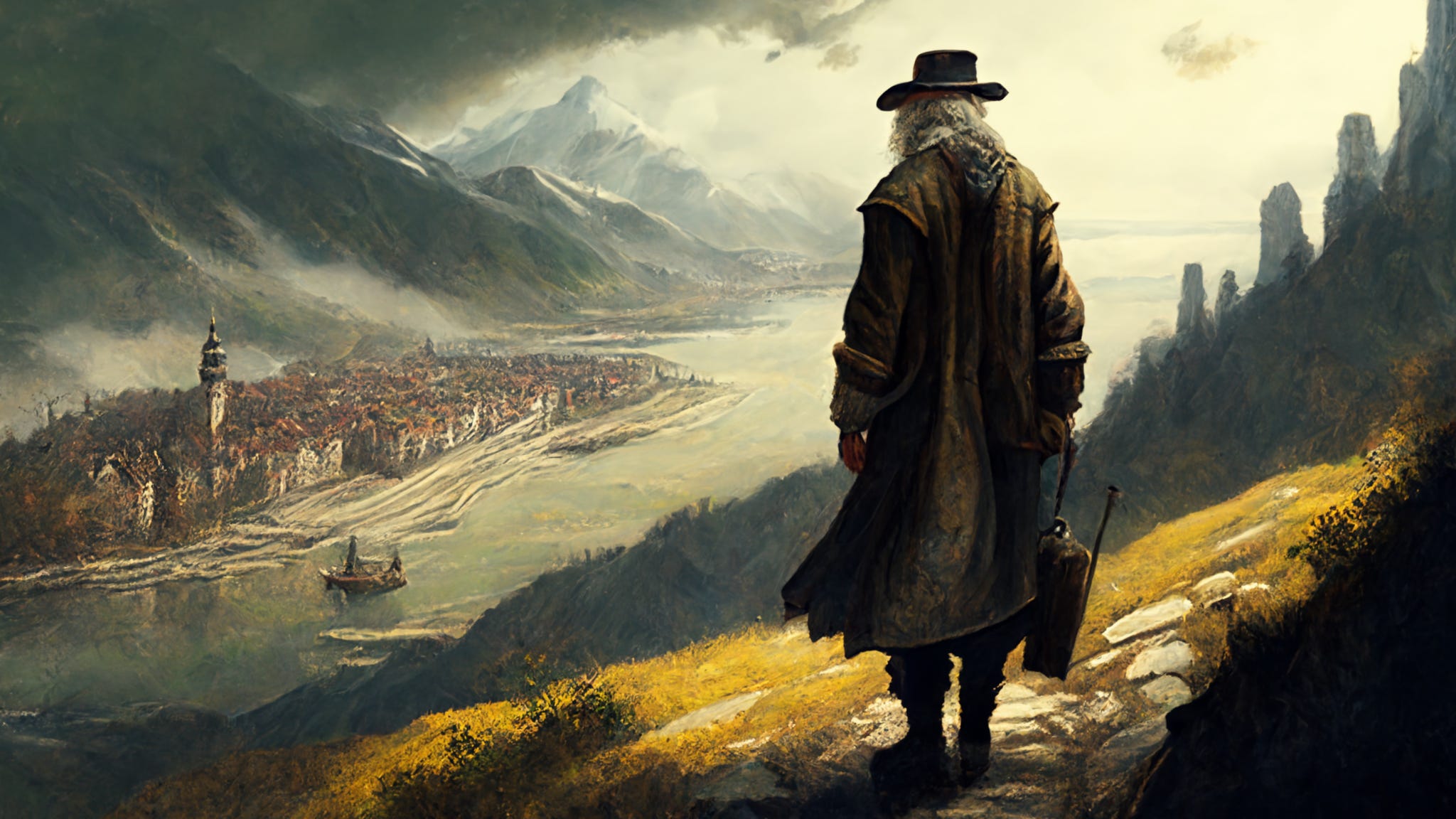
Wow, super easy. Isn’t it? So what are you waiting for?
This is great news for everyone building products and wanting to use data to optimize them - there is just one kind of analysis you need to master - the bad news is quite a steep hill to climb.
That might be why so few teams use retention analytics extensively to learn about their product activation and adoption.
So, let’s change this. My goal is quite a tough one - when you have finished this guide, you should have learned enough to open an analytics tool of your choice and create a retention rate that fits your product, telling you how you perform for product activation and adoption.
It might be a good idea to take the concept of retention apart as a start.
Thanks for reading the hipster data stack! Subscribe for free to receive new posts and support my work.
What is retention?
Most straightforwardly - someone comes back.
This means there needs to be a first time and then at least a second time. And maybe more return for something we can identify.
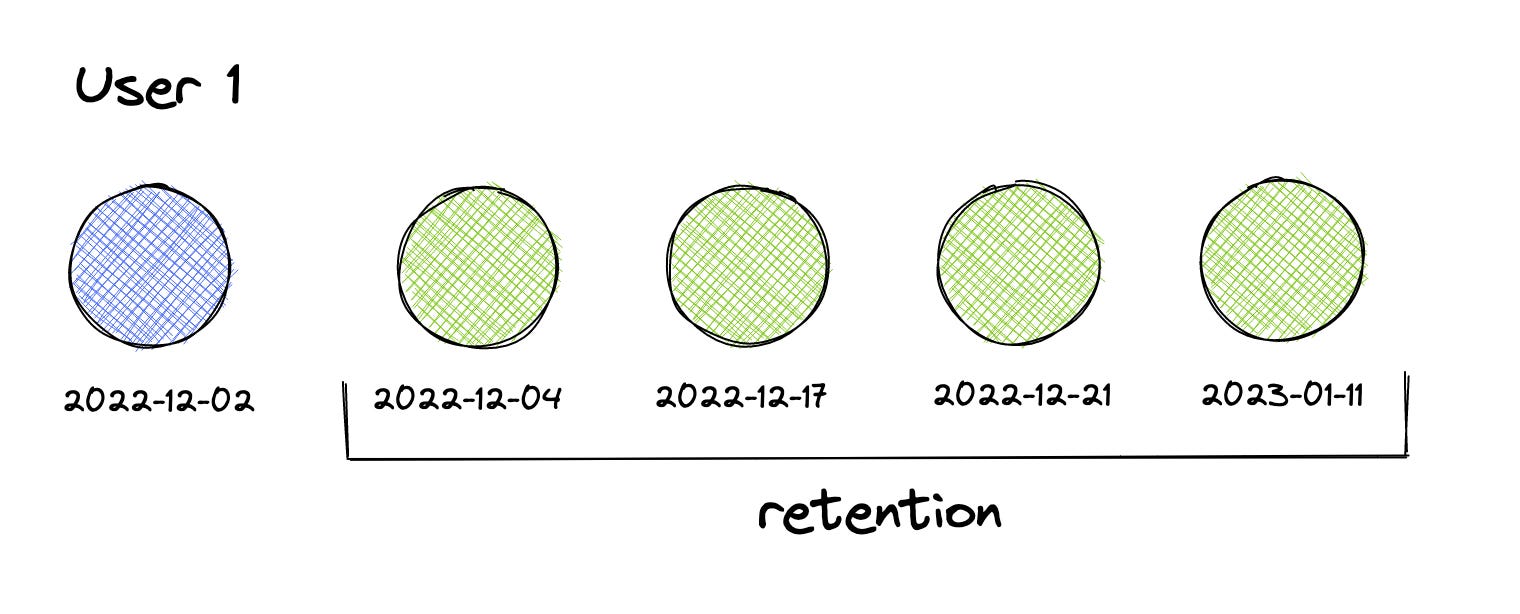
What does this identify mean?
To measure if someone has returned, we must be sure it is the same person. Therefore, our first essential requirement for retention analytics is a solid user (or account) identification.
I usually only do retention analytics when the service requires a sign-in. Only by that can we collect good enough identified user data to analyze retention.
So what about the first time?
How do we define a first time, and is there even one first time?
Of course not; there are plenty of first times in a customer journey. So, we must decide which one to use for a retention analysis. As we see here, there will be plenty of different retention analyses - just by picking different first times.
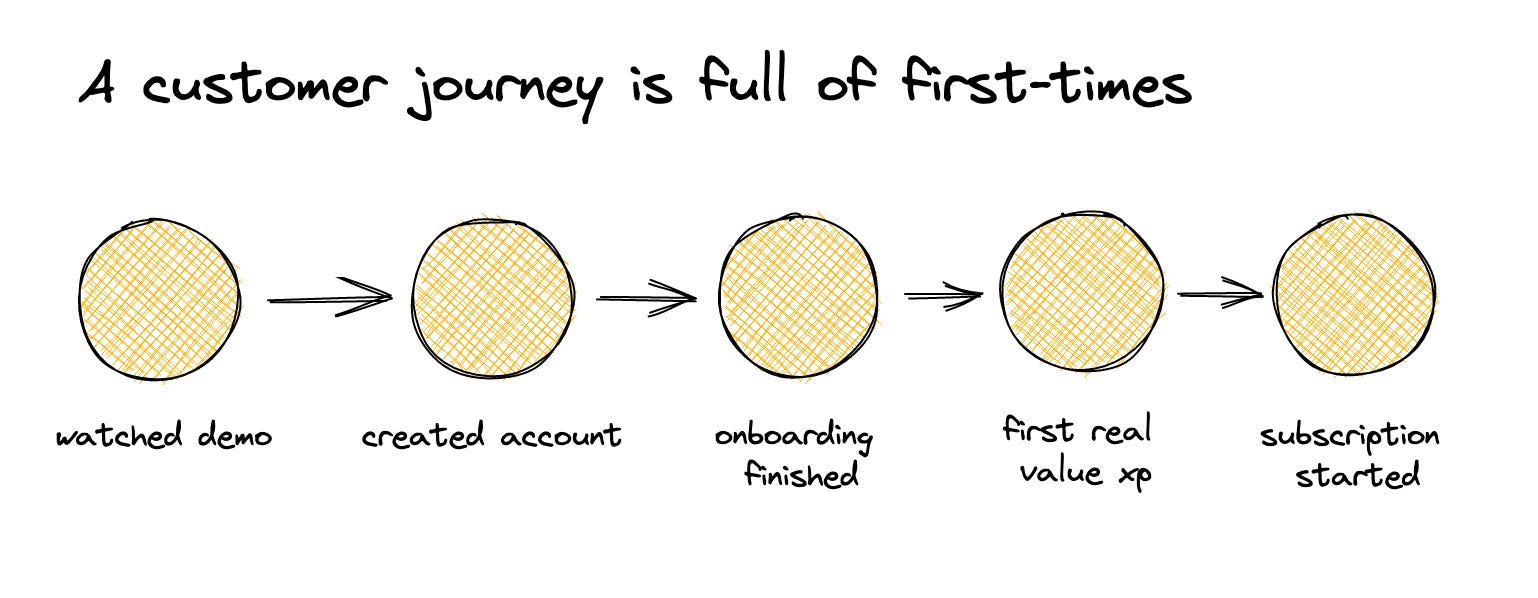
Good candidates to pick as a first time:
- Account created - the classic one and a good point to build your first retention; that is where everything starts (or at least where we indeed can track it and assign it to one identical account)
- Subscription started - another classic one, especially for revenue retention
- First value experience - a lot more tricky since you first have to create an event where a user has experienced a first value from your service. This varies naturally based on your service/product. In an analytics tool, it could be the first chart shared, in a todo app, the first todo completed.
But this retention can then be compared with the Account created one and therefore identify the difference and uplift it brings when you get users to experience a first value return.
Now the coming back
After you get your users to start something in your service, you need them to return.
Because as we all know, Acquisition > Activation > RETENTION > REVENUE. And we want to get to revenue eventually.
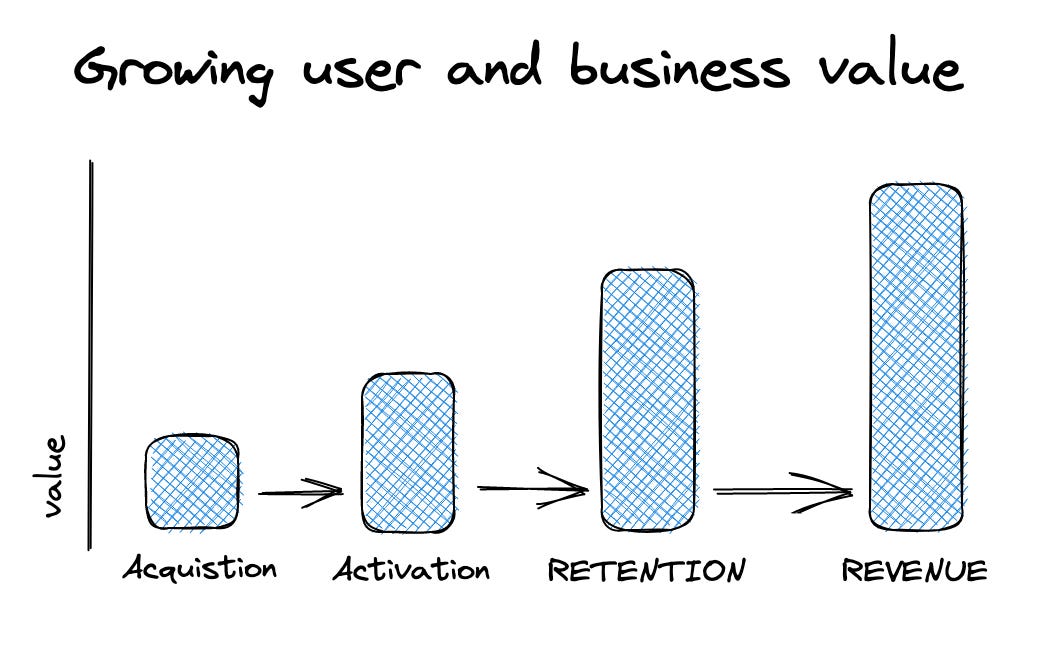
We need to define the “coming back condition,” which is often a specific event, but in some cases, it can also be a combination of events or several events.
We use this “coming back condition” to analyze it over a more extended period. Because we are primarily interested to see if people are returning regularly and doing something we hope they do.
What are good coming back conditions?
- for the simplest retention, we used account creation as a first-time condition; in combination, logging in or authenticated (if you have a longer authentication cookie lifetime) or just any event is a good start. By that, we are simply interested in attracting users to return. This is fine for a start, but it doesn’t tell us if they get value out of the product
- so the next one would extend the first-value example by simply taking the same value condition (see above) and using it as a “coming back condition.”
- In the subscription context, it is more straightforward; this would be the subscription renewal as a “coming back condition.”
How do we measure retention?
Now we have a “first-time” and a “coming back” condition. How do we calculate our retention? We can do this with a simple funnel and conversion rate.
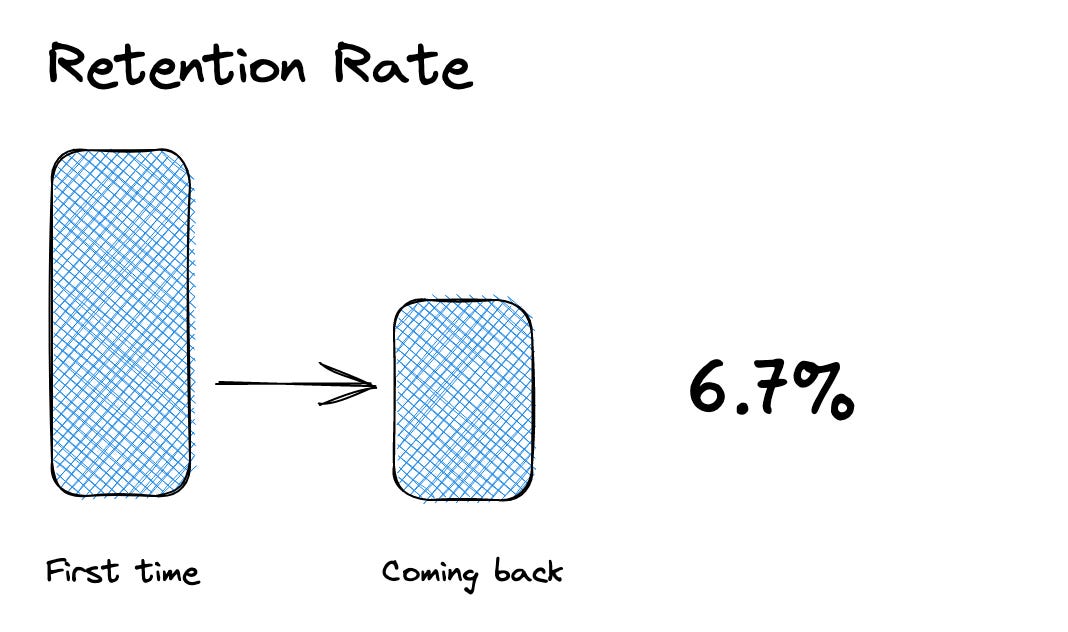
So we take all users that fulfill the "first-time" condition as the foundation and divide all users that satisfy the "coming back" condition by it. Which gives us a conversion rate. Quite good and easy. But you will quickly see that this is missing an essential piece that defines retention:
The time
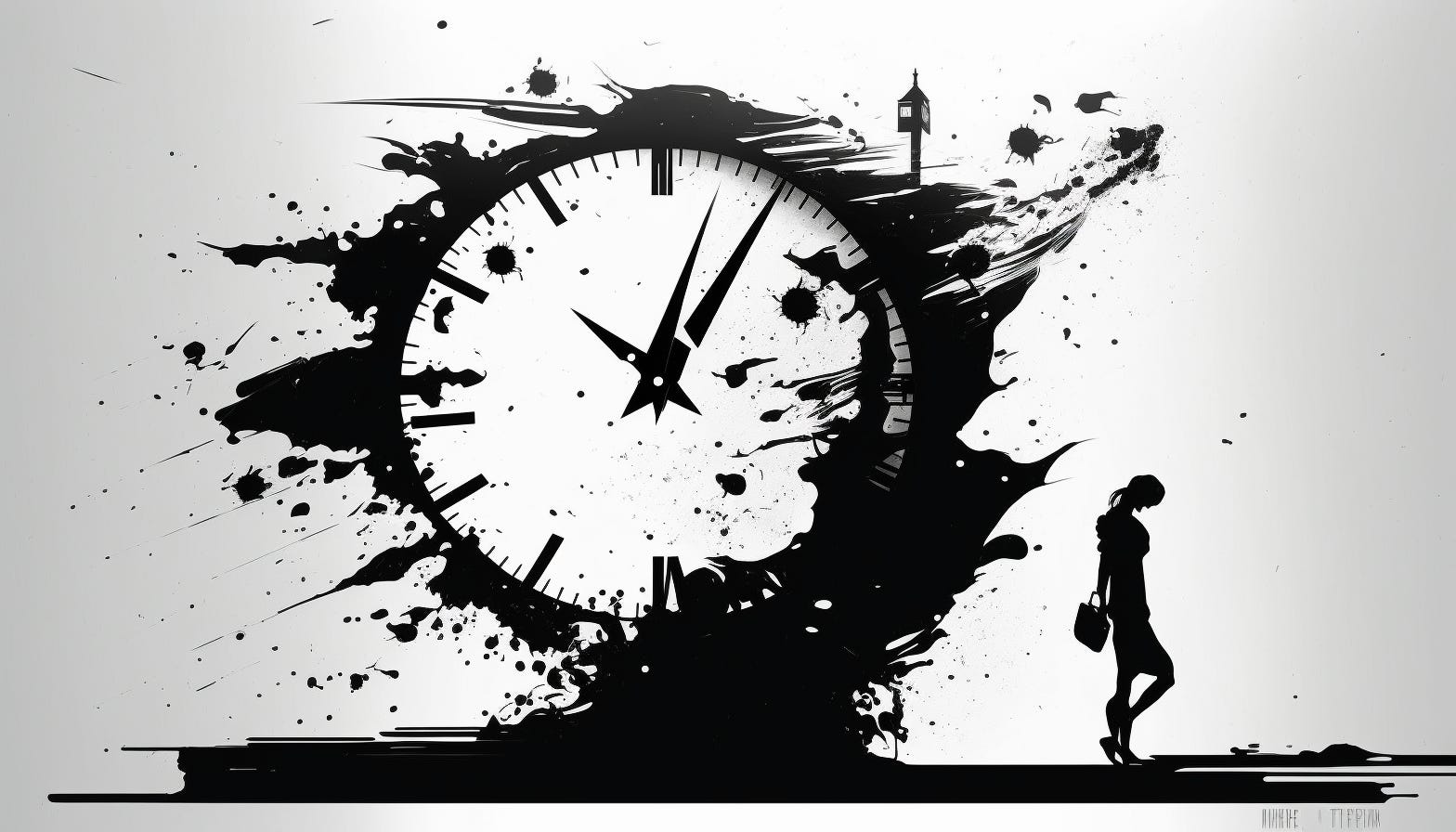
Looking from a high aggregated level on a conversion rate for retention does not tell us enough, and it’s too blended and generic because we don’t incorporate the time aspects and want to see if people are retained over time and not just in general.
How do we get time into this picture?
Measuring Retention with Cohorts
One type of analysis lets us investigate the performance from a first-time event to a "coming back" event over a defined time: a cohort analysis.
And it gives us an analysis over time and a way to analyze how the performance improves or decreases with the release of new features.
That is quite a package - let's unpack it.
We keep our "first-time" condition or event. And we also maintain our "coming-back" condition.
Now we need to define how we build a cohort. A cohort is a group of users who share the same criteria.
The most commonly used criteria in a cohort analysis are time, whether the day, week or month defines a cohort.
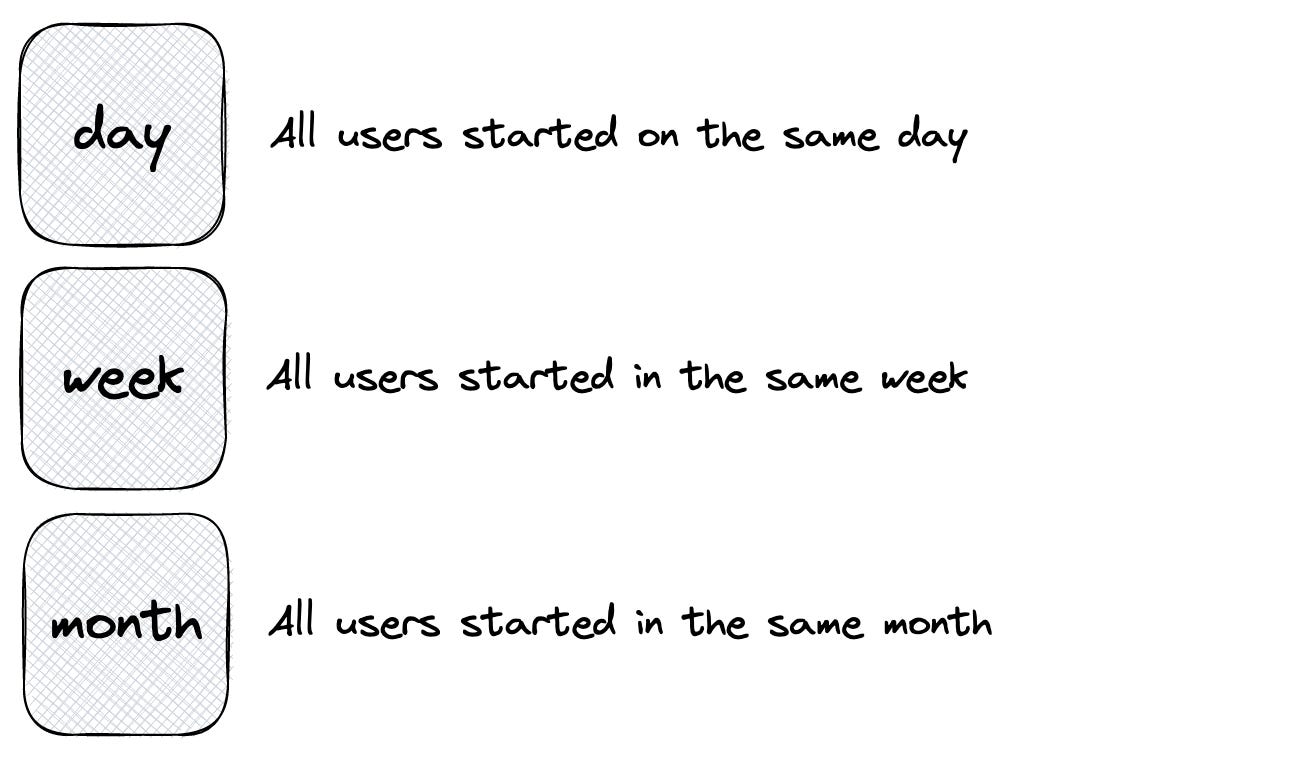
Why is this helpful? It helps us to quickly see if changes for our product are starting to increase or if the coming-back ratio is.
Here is a visualization of week cohorts:
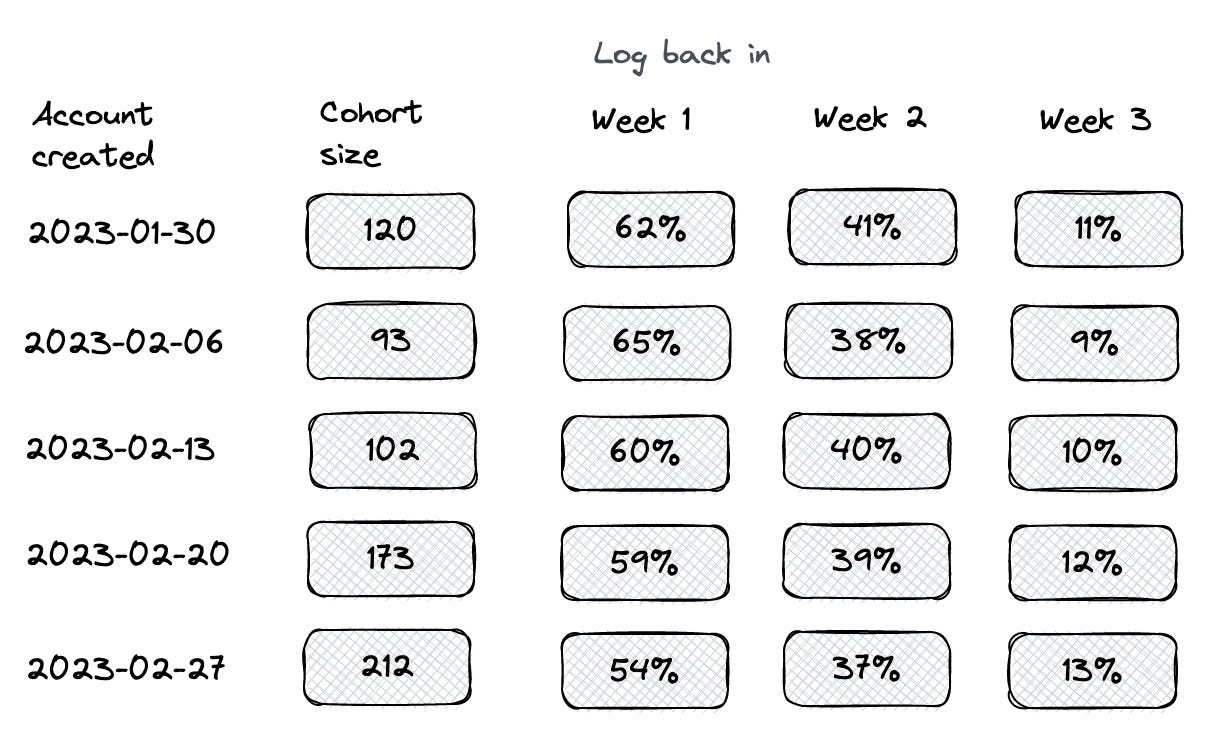
All the cohorts are in the left column and contain all users that have signed up for this specific week. The coming back percentage is the number of users logging in divided by all users in the cohort on a weekly base (in the next seven days and so on).
The table view of the cohort analysis immediately gives us feedback if we improve our retention. How does it do it?
Let's assume we want to improve retention for the first week (the first seven days). On average, over the last 12 weeks, we have had a first-week retention rate of 60%. The team worked hard to find two new ways to onboard our users, and they deployed feature 1 three weeks ago and feature 2 last week.
Our cohort table now looks like this:
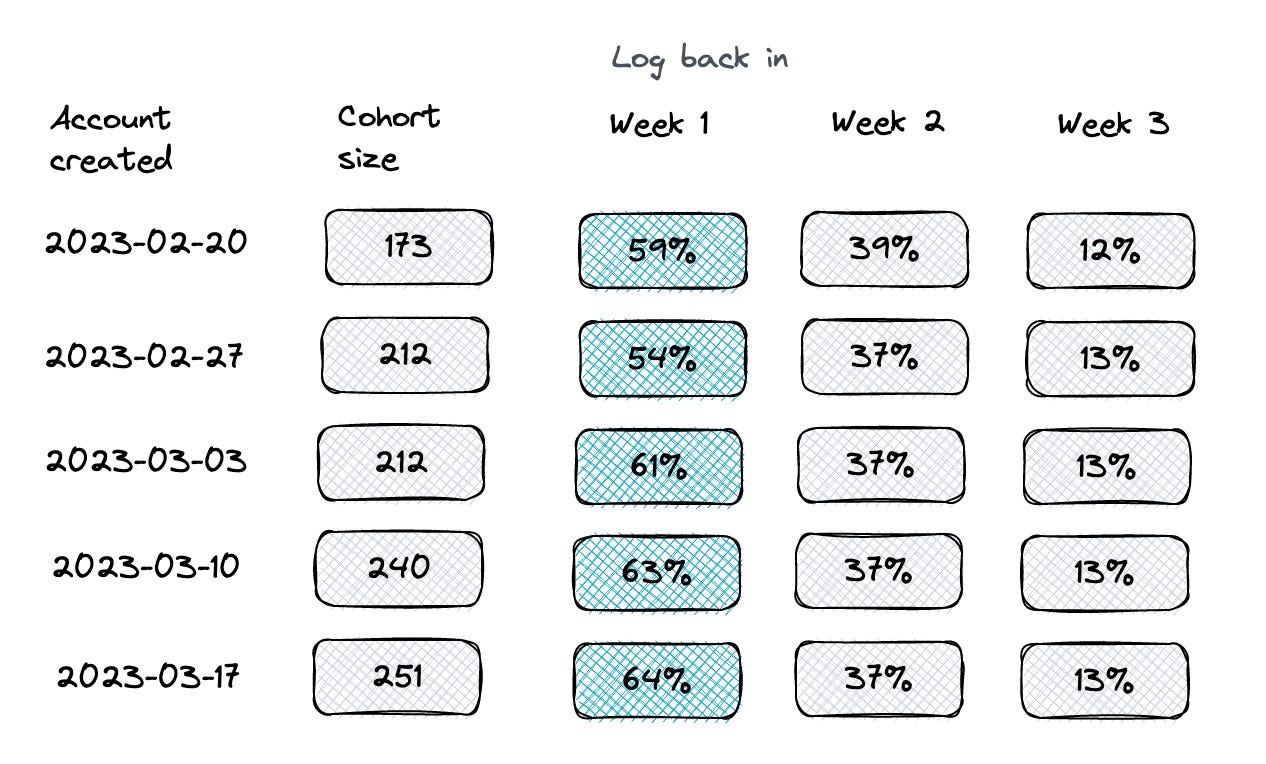
We can see that the first-week retention started to improve two weeks ago - which looks like a good indicator for feature 1. For feature 2, we need more data to come in. But as you can see here, we only need to watch each new cohort's first-week column to see if our efforts move any needle.
It is an excellent approach to test new features with a/b testing, but cohort analysis gives you a second option and a view of the general performance.
Going beyond the time-based cohorts
Defining different cohorts allows you to look at retention differently to find over and under-performing cohorts.
Here are the cohorts I usually create for a product:
- based on customer/subscription type - at least free vs. paid (if you have a free plan)
- based on the initial marketing channel that brought the signup
- based on subscription plans
- based on the platform used - if you offer multi-platform products
- based on feature usage
- based on company size
- based on the target audience (if defined and you track the data to identify them)
How to work regularly with retention data?
First, define your 2-3 core retention tables, like free vs. paid users, and put them on a dashboard. If you have a subscription model, add one for each plan.
If you start, start with people just coming back as come back criteria. You can advance from here to define how they get value from your product and use this as a comeback criterion.
Then define which period makes the most sense for these. Ideally, you can do this based on when you expect the users to return. In most products, this is on a weekly basis, and for subscription retention, it is most often on a monthly basis.
Now check the retention tables and see which retention rate makes the most sense as a focus metric. Pick the one where the drop is most significant. When you are starting, this is usually already the first seven days.
Now you can slice and dice with different cohorts to see if cohorts significantly perform better or worse than the general retention rate.
And then comes the fun part - work with the team to move the retention rate and always keep in mind:
- better retention for free users -> higher chance of subscription conversion
- better retention for paid users -> lower chance of churn
Sounds quite good to me - your way in product to become a revenue hero.
There are more details about retention analytics - so I will extend this guide over time. Make sure to subscribe to get the updates.
Topics to cover:
- extensive funnel/retention tables
- forecasting with cohorts
- rolling or fixed definitions
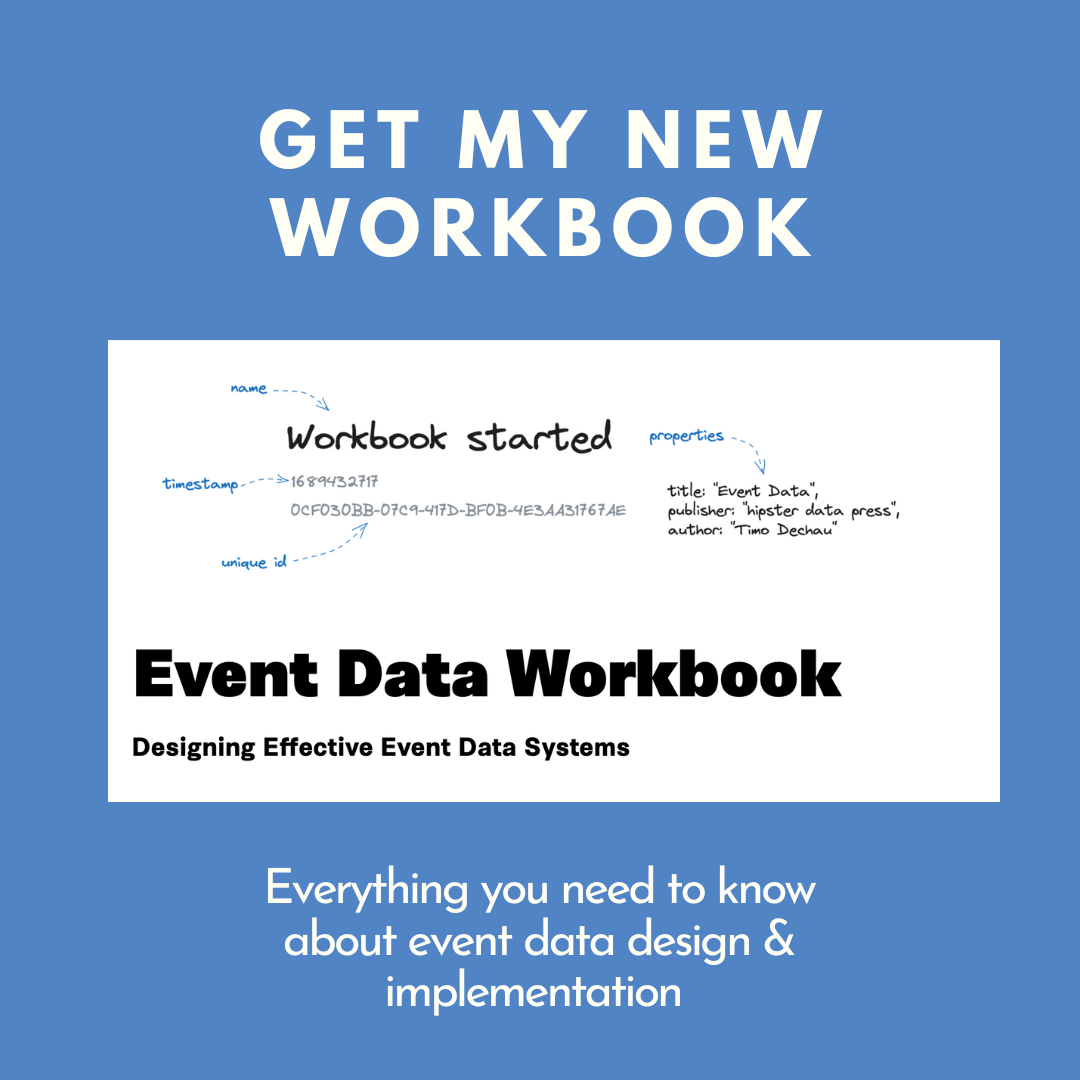
Thanks for reading the hipster data stack! Subscribe for free to receive new posts and support my work.